Analyzing Environmental Dynamics in the Center of Iran: Impacts of Climatic and Environmental Factors Using Remote Sensing and Spatial Analysis in Google Earth Engine and R Software
Mohamad Ali Ghoveh Nodoushan 1, Ali Akbar Jamali 2*, Seyed Masood Monavari 3 ,
Seyed Abolghasem Mirhoseini 1 , Mehdi Dehghani Zahedani 1
1 Department of Environment, Ya.C., Islamic Azad University, Yazd, Iran.
2 Department of GIS-RS and Watershed Management, May.C., Islamic Azad University, Maybod, Iran.
3 Department of Environmental Science, SR.C., Islamic Azad University, Tehran, Iran.
A R T I C L E I N F O |
|
ABSTRACT |
ORIGINAL ARTICLE |
|
Introduction: Environmental changes driven by anthropogenic and natural factors significantly affect human health, the environment, and economic systems globally. This study investigated the ecological impacts of environmental variables in the Yazd-Ardakan Plain using remote sensing data and geospatial analysis.
Materials and Methods: Google Earth Engine (GEE) and R software were used to assess spatiotemporal trends from 2018 to 2023 using data from Landsat-8, Sentinel-2, and Sentinel-5P. Key indices, including the Normalized Difference Vegetation Index (NDVI), temperature, surface runoff, and concentrations of NO2, CO, O3, and SO2, were calculated to evaluate patterns in green space, air quality, temperature, and precipitation.
Results: Elevated NO2 and CO levels were observed in the centers of Yazd and Ardakan, with O3 and SO2 peaking in 2019-2020 and improving by 2023. The highest aerosol concentrations were in 2019 and 2023, with a decrease in 2022. NDVI values peaked in 2020 but declined until 2023. The spatial analysis identified denser green spaces in Ardakan, elevated temperatures in Steel, and consistent precipitation across the regions. A positive correlation was found between temperature and pollution, whereas NDVI and precipitation showed negative correlations with temperature, indicating that vegetation loss exacerbates the effects of warming and pollution.
Conclusion: This study highlights significant climatic and environmental changes in the Yazd-Ardakan plain from 2018 to 2023, including rising temperatures and pollution, alongside declining precipitation and vegetation. These trends underscore the need for targeted management strategies to mitigate environmental degradation, protect public health, and promote sustainable development through advanced monitoring and policy intervention. |
Article History:
Received: 17 November 2024
Accepted: 20 February 2025
|
|
*Corresponding Author:
Ali Akbar Jamali
Email:
aa.jamali@iau.ac.ir
Tel:
+98 3531872762 |
|
Keywords:
Remote Sensing Technology,
Climate Change,
Global Warming,
Air Pollution,
Yazd City,
Iran. |
Citation: Ghoveh Nodoushan MA, Jamali AA, Monavari SM, et al. Analyzing Environmental Dynamics in the Center of Iran: Impacts of Climatic and Environmental Factors Using Remote Sensing and Spatial Analysis in Google Earth Engine and R Software. J Environ Health Sustain Dev. 2025; 10(1): 2551-67.
Introduction
Since the early 20th century, industrialization has played a crucial role in economic development, taking various forms, such as industrial complexes, zones, and hubs 1. Proper land-use planning and labor distribution are essential for minimizing environmental impact while maximizing economic benefits. However, industrial activities release air pollutants such as particulate matter (PM10 and PM2.5), sulfur oxides (SOx), nitrogen oxides (NOx), volatile organic compounds (VOCs), and carbon oxides, posing significant environmental and health risks 2. Although industries are crucial for economic growth, improper planning exacerbates urban pollution and worsens air quality 3, 4.
Climate change and air pollution are pressing global challenges that require urgent attention. Rising greenhouse gas emissions and surface temperatures contribute to extreme weather events, such as heatwaves, wildfires, floods, droughts, and storms 5. These changes directly and indirectly affect human health by increasing exposure to allergens and pollutants 6. Meteorological factors, including temperature, humidity, wind, and atmospheric mixing, affect pollutant dispersion, dilution, chemical transformation, and deposition 7. Climate change is expected to exacerbate air pollution in densely populated areas by altering ventilation, precipitation, and pollutant removal processes 8.
Remote sensing is an advanced tool for environmental monitoring that enables the collection and analysis of Earth surface data using satellite sensors. Google Earth Engine (GEE) has emerged as a powerful platform for visualizing and analyzing climate and environmental variables such as temperature, precipitation, vegetation, and land cover 9-11. Several studies have highlighted the effectiveness of remote sensing and GEE in environmental studies. For instance, Moradi et al. (2024) developed predictive models for air pollution in Yazd, Iran, using meteorological and pollutant data 12. Ghorbanian et al. (2021) mapped mangrove ecosystems in Qeshm, Iran, using Sentinel-1 and Sentinel-2 images with the random forest algorithm, achieving a high classification accuracy 13. Karam et al. (2018) analyzed land-use changes in Yazd-Ardakan plain over 30 years, observing a decline in desert areas and expansion of other land uses 14.
Other notable studies include Liu et al. (2023), who monitored long-term vegetation trends using the Normalized Difference Vegetation Index (NDVI) and Landsat imagery on Zhoushan Island, China, and showed a decline in vegetation health over time 15. Tabunschik et al. (2023) used Sentinel-5 satellite imagery and GEE to analyze air pollution in Crimean mountain river basins, identifying significant spatial and temporal variations in NO₂ 16. Xing et al. (2022) examined air quality changes in Shandong Province, China, before and after COVID-19 lockdowns, revealing substantial decreases in CO and NO₂ due to reduced human activity 17.
Climate and environmental changes in industrial regions, such as the Yazd-Ardakan plain, are driven by greenhouse gas emissions, land-use changes, and industrial activities. These changes affect biodiversity, economic stability, and the public health. Rising temperatures, increased rainfall variability, and air pollution in Yazd-Ardakan necessitate improved environmental management to address the challenges related to water scarcity, industrial expansion, and urbanization. Given the ecological complexity of the region, comprehensive spatial modeling is required to effectively assess and predict environmental and climatic transformations.
This study aimed to model climate-environment interactions in the Yazd-Ardakan region using remote sensing data, Java coding, artificial intelligence, and R-based statistical analysis on the GEE platform. By analyzing spatiotemporal environmental changes, this study provides valuable insights into sustainable environmental management. This study hypothesizes that (1) temperature and atmospheric pollution levels have increased, whereas precipitation and vegetation cover have declined, and (2) these environmental changes significantly affect regional climate stability and overall environmental quality.
Despite the growing body of literature on climate-environment interactions, there is a notable lack of spatial modeling studies on the Yazd-Ardakan plain. This study fills a critical gap by integrating advanced remote sensing, programming, and statistical techniques to analyze time-series trends and environmental changes. Such an approach is crucial for developing effective environmental policies and management strategies in under-researched regions such as Yazd-Ardakan.
Materials and Methods
Study Area
The present study encompassed all industrial parks in the Ardakan-Yazd plain. This region is geographically located between 31°47'31" to 32°13'32" North latitude and 53°40'53" to 54°27'54" East longitude, falling within UTM zone 39. The Ardakan-Yazd plain is a significant industrial hub in Iran, characterized by an arid climate, sparse vegetation, and diverse industrial activities. This area faces various environmental challenges, including water scarcity, air pollution, and climatic variability, making it an ideal location for studying the interactions between industrial activities, environmental changes and climatic trends (Figure 1).
.JPG)
Figure 1: Study area location in Iran and Yazd province
The study area, the Ardakan-Yazd plain, has an arid climate characterized by hot summers, cold winters, and low annual rainfall. Industrial activities, including metal production, ceramics, textiles, and mining, significantly contribute to air and water pollution and land degradation. The region has predominantly flat topography, with mountainous areas in the north and east, which affects local climatic conditions and air pollutant dispersion patterns. Understanding these dynamics is crucial for developing sustainable industrial practices and mitigating their environmental impacts.
A descriptive analysis of air pollutant levels from 2018 to 2023 (Figure 2) across various regions—Steel, Yazd, Control, Rostagh, Saduq, JahanAbad, Meybod, HasanAbad, and Ardakan—revealed significant spatial variation. The highest NO₂ levels (Figure 2A) were in the Steel and Yazd regions, indicating industrial and urban emissions, whereas the control area had the lowest levels. The highest aerosol concentrations (Figure 2B) were in the control region, with Steel and Yazd also showing elevated levels, suggesting localized pollution sources. CO concentrations (Figure 2C) were the highest in the Steel and control areas, with Rostagh recording the lowest values. O₃ levels (Figure 2D) were relatively uniform, with a slight increase in the control region, potentially due to local emission patterns. SO₂ levels (Figure 2E) peaked in the Steel region, with notable variability in Saduq, indicating significant industrial emissions.
These findings highlight the need for targeted air quality management strategies, particularly in industrial and urban areas where the highest pollutant levels were observed. Addressing pollution sources and implementing mitigation measures are essential for improving overall air quality and public health.
The standard level of air pollution factors can be compared with the existing pollution for some decisions. Table 1 presents these standards.
.JPG)
Figure 2: Descriptive analysis of air pollutants in Yazd and surrounding regions (A: NO2, B: Aerosol, C: CO, D: O3, E: SO2 mean of 2018 to 2023) (units: molecules per cm³) (https://www.esa.int/Applications/ Observing_ the_Earth/ Copernicus/Sentinel-5P/Sentinel-5P_brings_air_pollution_into_focus, access date 1 March 2025)
Table 1: Standard level of air pollution factors
Pollutant |
Concentration |
Averaging
period |
Legal nature |
Permitted
exceedences
each year |
Fine particles (PM2.5) |
25 µg/m3 |
1 year |
Target value to be met as of 1.1.2010
Limit value to be met as of 1.1.2015 |
n/a |
Fine particles (PM2.5) |
20 µg/m3 |
1 year |
Stage 2 limit value to be met as of 1.1.2020 *** |
n/a |
Sulphur dioxide (SO2) |
350 µg/m3 |
1 hour |
Limit value to be met as of 1.1.2005 |
24 |
Sulphur dioxide (SO2) |
125 µg/m3 |
24 hours |
Limit value to be met as of 1.1.2005 |
3 |
Nitrogen dioxide (NO2) |
200 µg/m3 |
1 hour |
Limit value to be met as of 1.1.2010 |
18 |
Nitrogen dioxide (NO2) |
40 µg/m3 |
1 year |
Limit value to be met as of 1.1.2010 * |
n/a |
Particulate matter (PM10) |
50 µg/m3 |
24 hours |
Limit value to be met as of 1.1.2005 ** |
35 |
Particulate matter (PM10) |
40 µg/m3 |
1 year |
Limit value to be met as of 1.1.2005 ** |
n/a |
Carbon monoxide (CO) |
10 mg/m3 |
Maximum daily 8 hour mean |
Limit value to be met as of 1.1.2005 |
n/a |
Ozone |
120 µg/m3 |
Maximum daily 8 hour mean |
Target value to be met as of 1.1.2010 |
25 days averaged over 3 years |
(https://environment.ec.europa.eu/topics/air/air-quality/eu-air-quality-standards_en)
Descriptive Analysis of Environmental Variables in Yazd and Surrounding Regions (2018-2023)
The descriptive analysis of environmental variables in Yazd and its surrounding regions from 2018 to 2023 (Figure 3) revealed significant spatial variations. The highest NDVI values (Figure 3A) were observed in Ardakan and HasanAbad, suggesting better vegetation health and more favorable conditions for plant growth. Soil temperature (Figure 3B) peaked in the control region, followed by Steel and Yazd, indicating potential influences from industrial heat or land use practices.
Surface runoff (Figure 3C) was significantly higher in the Steel region, suggesting potential water management challenges and increased soil erosion due to industrial activities or land-use changes. The air temperature at 2 m (Figure 3D) showed slightly higher values in the Steel, Yazd, and control regions, likely affected by urban heat island effects. The highest wind speed (Figure 3E) was observed in the control region, indicating potential effects on local climate and pollution dispersion. Total precipitation (Figure 3F) was slightly higher in the control and HasanAbad regions, although the overall differences were minor, suggesting localized variations in water availability.
These findings emphasize the interplay between natural and human-induced factors in shaping environmental conditions. Addressing elevated runoff, localized temperature increases, and wind-driven pollution dispersion is essential for improving regional environmental management and ensuring sustainability.
.JPG)
Figure 3: Spatial distribution of environmental variables in Yazd and surrounding regions (A: NDVI, B: Soil temperature 0C, C: Surface runoff mm, D: Temperature in 2m, -0C, E: Wind speed m/s, F: Total annual precipitation, mean of 2018 to 2023)
Workflow Diagram
This section presents a workflow diagram illustrating the sequential steps and methodologies employed throughout the study, depicting the systematic approach used for data collection, analysis, and interpretation. The workflow includes the integration of remote sensing data, application of advanced analytical tools, and utilization of statistical methods to achieve the study objectives (Figure 4).
This workflow diagram provides a clear and systematic representation of the study approach, ensuring the transparency and reproducibility of the research process. This highlights the comprehensive nature of the study, which combines remote sensing, statistical analysis, and advanced computational tools to address the complex interactions between industrial activities, environmental changes, and climatic trends in the Ardakan-Yazd plain.
.JPG)
Figure 4: Flowchart of the research process
Data Collection
Remote sensing data acquisition and characteristics
This study utilized remote sensing data and techniques through the GEE platform and R software for an in-depth spatiotemporal analysis of environmental and climatic dynamics in the Yazd-Ardakan region from 2018 to 2023. This study employed time-series data from Sentinel-2, Sentinel-5P, and Landsat-8 satellites to capture and analyze various environmental variables. The integration of these datasets via the GEE platform facilitated a detailed examination and statistical analysis, as summarized in Table 2.
Table 2: Details of satellite images
Satellite |
Sensor |
Spatial Resolution |
Source |
Sentinel-2 |
C-SAR Multi-Spectral Instrument (MSI) |
10 m, 20 m, 60 m |
European Space Agency (ESA) |
Sentinel-5P |
TROPOMI |
7 x 3.5 km |
ESA |
Landsat-8 |
Operational Land Imager(OLI) and Thermal Infrared Sensor (TIRS) |
30 meters (OLI), 100 meters (TIRS) |
United States Geological Survey (USGS) |
Overview and Application of Remote Sensing and Analytical Tools
Sentinel-2 is a polar-orbiting satellite developed by the ESA under the Copernicus program. It carries a MultiSpectral Instrument (MSI) that captures images across 13 spectral bands with spatial resolutions of 10 m (visible and near-infrared), 20 m (red edge and shortwave infrared), and 60 m (atmospheric correction) 18, 19. This study utilized Sentinel-2 data to compute the NDVI, a key indicator of vegetation health and land cover changes 20, 21.
Sentinel-5P, developed by the ESA in collaboration with the Netherlands, is equipped with the TROPOspheric Monitoring Instrument (TROPOMI) for atmospheric composition analysis. It measures air pollutants, such as NO₂, CO, O₃, SO₂, and aerosols, providing essential data on air quality 22. This study employed Sentinel-5P Level 2 (L2) data to analyze the tropospheric pollution trends.
Landsat-8, operated by the USGS and NASA, includes the OLI and TIRS with spatial resolutions of 30 m and 100 m, respectively 23. It was used to analyze the temperature, wind speed, surface runoff, and precipitation in Yazd-Ardakan plain.
Analytical Tools: R software facilitated statistical analyses and data visualization, whereas GEE enabled large-scale geospatial data processing. These tools are instrumental in examining environmental trends and generating insights. More details on GEE can be accessed at https://earthengine.google.com/ (access date: 12 Feb 2025).
Results
Comprehensive Comparison of NO₂ Pollution in Yazd and Surrounding Areas (2018–2023)
The NO₂ figures for Yazd-Ardakan plain from 2018 to 2023 (Figure 5) are presented in six sections (A–F), each corresponding to a specific year. Dark blue and green indicate lower NO₂ concentrations, whereas light blue, yellow, and red indicate higher concentrations. Black spots indicate industrial estate locations.
In 2018 (A), NO₂ levels were low across most of the plain, with moderate concentrations in central areas, including Atrabad, Ahmadabad, and Meybod. Western regions like Aqda, Nedoushan, and Sadrabad had very low concentrations. In 2019 (B), NO₂ levels increased significantly, with Yazd, Meybod, and Zarch turning red to yellow, indicating industrial influence. This trend continued in 2020 (C), with concentrations peaking in the central and southern areas, particularly in Yazd, Meybod, Zarch, and Atrabad.
In 2021 (D), NO₂ levels remained high in the central regions but decreased in the western areas. By 2022 (E), pollutant levels slightly declined, possibly because of control measures. However, in 2023 (F), the NO₂ levels rebounded in some central and southern areas.
Overall, NO₂ pollution in the Yazd-Ardakan plain increased from 2018 to 2023, peaking in 2020. The central regions, particularly Meybod and Zarch, consistently experienced the highest concentrations, highlighting the urgent need for air quality management.
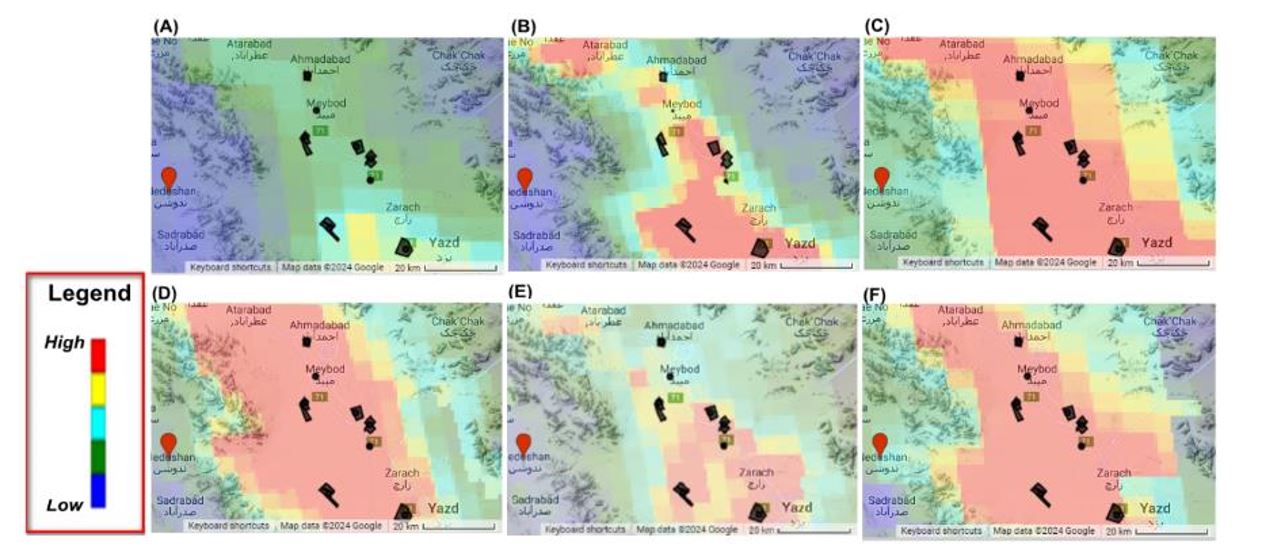
Figure 5: Changes in NO2 pollution levels in Yazd and surrounding areas (A to F: 2018-2023)
Comprehensive Comparison of Aerosol Pollution in Yazd and Surrounding Areas (2018–2023)
The aerosol trends and distribution in Yazd-Ardakan Plain from 2018 to 2023 (Figure 6) are presented in six sections (A–F), corresponding to each year, as follows. Dark to light blue colors indicate lower aerosol concentrations, whereas red, brown, and black colors indicate higher concentrations. Black dots represent industrial estates.
In 2018 (A), aerosol concentrations were generally low, though central and western areas, including Yazd, Zarch, Meybod, and Ahmadabad, showed higher levels. In 2019 (B), aerosol concentrations decreased, with more areas displaying low levels, except around industrial estates and in the northern regions. By 2020 (C), aerosol concentrations further declined, with industrial estate areas showing reduced levels of aerosol concentrations.
In 2021 (D), aerosol levels remained similar to those in 2020, although they were slightly elevated around industrial estates. However, in 2022 (E), aerosol concentrations increased, particularly near Yazd and the industrial estates. By 2023 (F), aerosol levels increased significantly, with higher concentrations in the central and western regions, particularly around industrial estates.
Overall, aerosol concentrations decreased from 2018 to 2020, followed by an upward trend from 2021, peaking in 2023. The central regions, particularly near industrial estates such as Meybod and Ardakan, exhibited the highest concentrations, reflecting the impact of industrial activities on air quality.
Figure 6: Changes in aerosol pollution levels in Yazd and surrounding areas (A to F: 2018-2023)
Comprehensive Comparison of CO Pollution in Yazd and Surrounding Areas (2018–2023)
The CO distribution in the Yazd-Ardakan Plain from 2018 to 2023 (Figure 7) is shown in six sections (A–F), representing each year. Red and yellow indicate higher CO concentrations, whereas blue and black indicate lower levels. Black spots indicate industrial estates.
In 2018 (A), the CO levels were low across most areas, particularly in the central and western regions. Even around industrial estates, the concentrations remained low. In 2019 (B), no significant changes were observed, and low CO levels persisted in most areas. In 2020 (C), the pattern remained largely unchanged, with minor fluctuations near the industrial estates.
In 2021 (D), the CO concentrations remained low, with no substantial changes compared to the previous years. This trend continued in 2022 (E), with low concentrations across the region and no notable shifts. By 2023 (F), the situation remained stable, with no increase in CO pollution.
Overall, 2018 the 2023, Yazd-Ardakan Plain consistently experienced low CO levels from 2018 to 2023. The spatial pattern remained stable, with no significant variations, even in industrial estates. No critically high-CO areas were identified, confirming that CO pollution remained minimal throughout the study period.
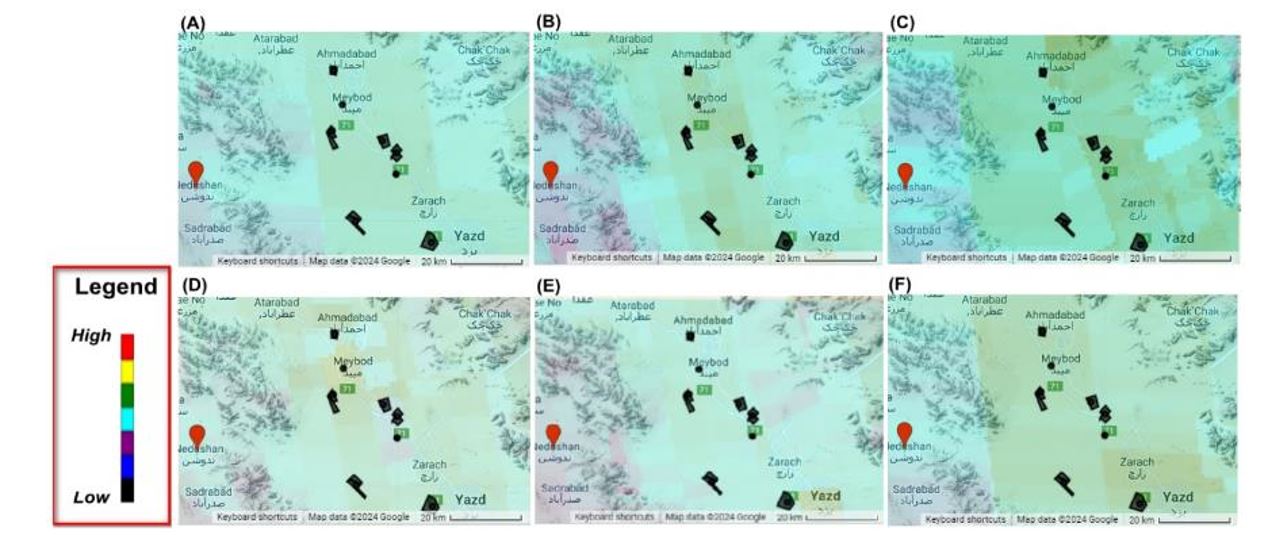
Figure 7: Changes in CO pollution levels in Yazd and surrounding areas (A to F: 2018-2023)
Comprehensive Comparison of O₃ Pollution in Yazd and Surrounding Areas (2018–2023)
The O₃ distribution in the Yazd-Ardakan Plain from 2018 to 2023 (Figure 8) is shown in six sections (A–F), representing each year. Red, green, and yellow indicate higher O₃ concentrations, whereas dark blue and black signify lower levels. Black spots indicate industrial estates.
In 2018 (A), O₃ levels were relatively high in the central and northern areas, especially near industrial estates, whereas the southern and eastern regions had lower concentrations. In 2019 (B), the O₃ concentrations increased and became more widespread, particularly in the central and northern areas. This trend continued in 2020 (C), with a sharp increase in O₃ levels across the entire plain, particularly in the north and center.
In 2021 (D), the O₃ concentrations remained high, particularly in the northern and eastern regions. In 2022 (E), the levels declined in the south but remained elevated in the central and northern areas. By 2023 (F), the O₃ concentrations peaked, affecting almost all the northern, central, and eastern regions.
Overall, O₃ levels showed a continuous increase from 2018 to 2023, particularly in the northern and central areas near industrial estates. The highest concentrations were observed in Meybod, Ardakan, and Zarch, indicating a strong link between industrial activity and pollution in these areas. The northern, central, and eastern sections were the most affected, highlighting
the impact of industrial emissions on air
quality.
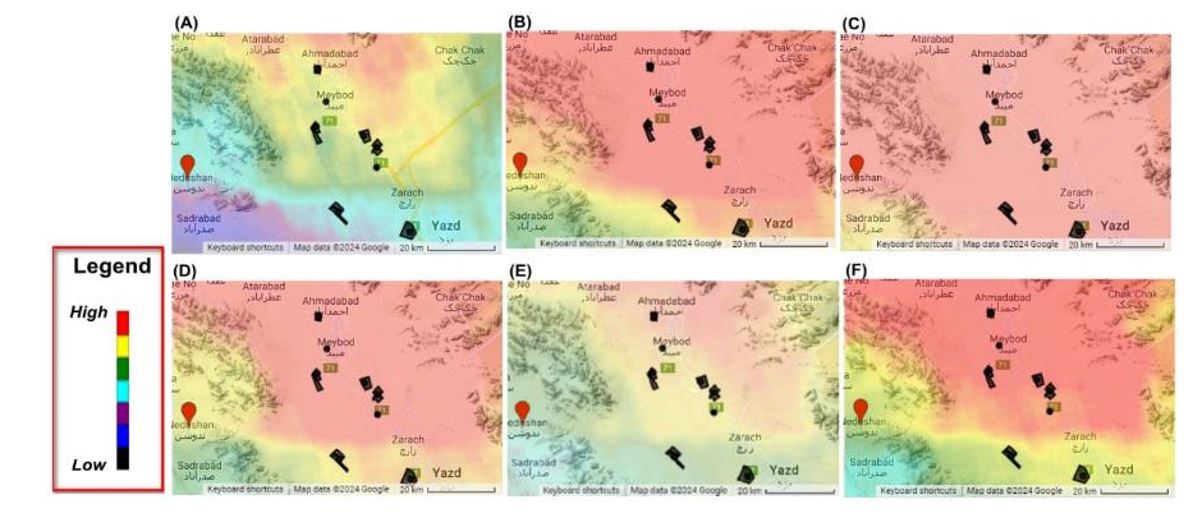
Figure 8: Changes in O3 pollution levels in Yazd and surrounding areas (A to F: 2018-2023)
Comprehensive Comparison of SO₂ Pollution in Yazd and Surrounding Areas (2018–2023)
The SO₂ distribution in the Yazd-Ardakan Plain from 2018 to 2023 (Figure 9) is shown in six sections (A–F), each representing a year. Red, yellow, and green indicate higher SO₂ concentrations, whereas blue and black signify lower levels. Black spots indicate industrial estates.
In 2018 (A), SO₂ concentrations were relatively low in the southern and eastern regions but were higher in the central and northern areas, particularly near industrial estates. In 2019 (B), SO₂ levels increased, especially in the central and northern areas, with red and yellow indicating higher concentrations. By 2020 (C), SO₂ dispersion had expanded, with significant increases in the central and northern areas, particularly near industrial estates.
In 2021 (D), SO₂ levels rose further, with the highest concentrations recorded near the industrial estates in Meybod and Ardakan. In 2022 (E), the concentrations decreased in the south and east but remained high in the central and northern areas. By 2023 (F), SO₂ concentrations peaked, with widespread red and yellow colors in the northern, central, and eastern regions, reflecting the continued impact of industrial activities.
Overall, SO₂ levels fluctuated, but industrial estates consistently showed high SO₂ concentrations. The most affected areas included Meybod, Ardakan, and Zarch, demonstrating a direct link between industrial activities and air pollution, with emissions having a significant effect on air quality.
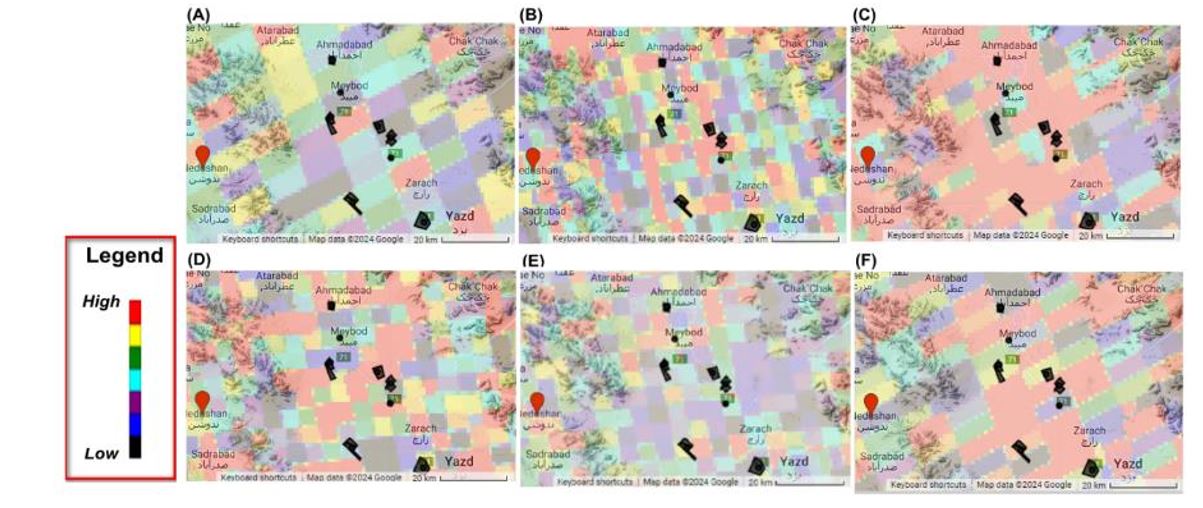
Figure 9: Changes in SO2 pollution levels in Yazd and surrounding areas (A to F: 2018-2023)
Temporal and Spatial Variations in NDVI for Yazd and Surrounding Areas (2018–2023)
A series of maps (Figure 10) illustrate the NDVI variations in Yazd from 2018 to 2023. In 2018 (A), NDVI values were low, indicating sparse vegetation, with slightly higher values in the northern and northwestern regions. In 2019 (B), NDVI increased, particularly in the central and southern areas, likely due to improved climatic conditions or agricultural activity.
By 2020 (C), NDVI peaked, especially in the western and southwestern areas, reflecting optimal vegetation growth, possibly due to favorable weather or increased water availability. In 2021 (D), NDVI declined, most notably in the eastern and southeastern parts, likely due to drought or environmental stress. In 2022 (E), a partial recovery occurred, particularly in the northeastern and central regions, indicating improved climatic conditions or successful interventions. By 2023 (F), the NDVI values stabilized, with moderate vegetation cover across the region. The northern areas continued to exhibit slightly higher NDVI values than the southern areas, reflecting variations in soil fertility, water availability, and land use.
Overall, NDVI trends highlight the effects of climate and environmental factors on vegetation cover. The northern and western regions consistently showed higher NDVI values, whereas the southern and eastern areas faced challenges owing to lower water availability. Continuous monitoring and effective management strategies are crucial for sustaining vegetation and agricultural health in Yazd, Iran.
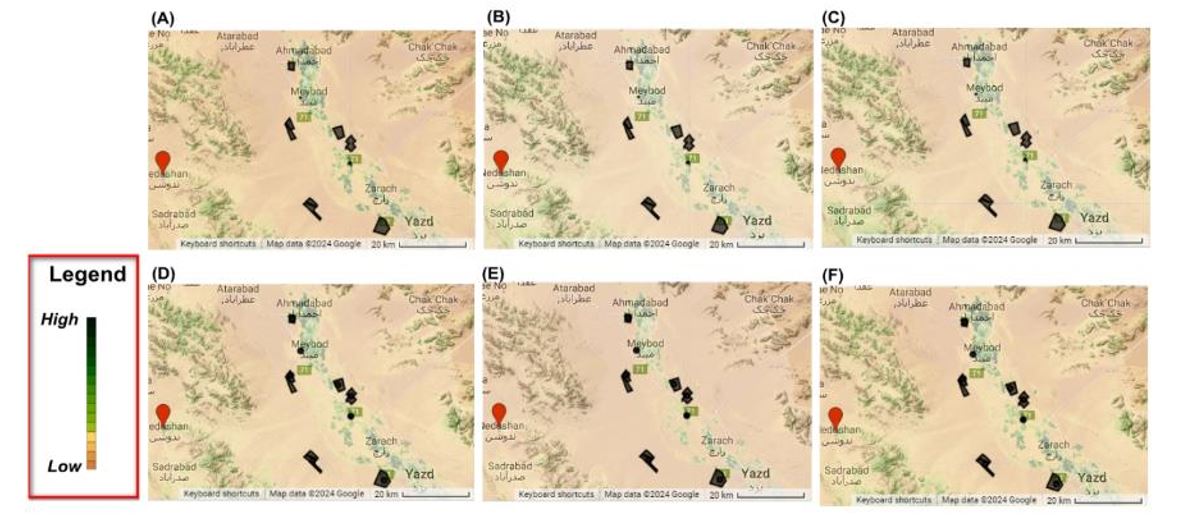
Figure10: Temporal and spatial variations in NDVI for Yazd and surrounding areas (A to F: 2018-2023)
One-Way ANOVA Analysis of Air Pollutants (2018–2023)
The one-way ANOVA results highlighted variations in air pollutant levels (NO₂, Aerosol, CO, O₃, and SO₂) from 2018 to 2023 (Figure 11).
NO₂ (A): The lowest levels were in 2018 (‘a’), peaked in 2021 and 2022 (‘e’ and ‘b’), then declined in 2023 (‘d’), indicating a pollution surge followed by a reduction.
Aerosol (B): Peaks were observed in 2018 and 2023 (‘a’ and ‘d’), while the lowest levels occurred in 2020 and 2021 (‘c’ and ‘e’), showing fluctuations in the data.
CO (C): No significant differences were observed, suggesting stable CO concentrations.
O₃ (D): The median values remained consistent, indicating minimal variation.
SO₂ (E): The highest levels appeared in 2018 and 2019 (‘a’ and ‘e’), followed by a notable decline in 2022 and 2023 (‘b’ and ‘d’), reflecting effective pollution control measures.
NO₂ and aerosol levels fluctuated, CO and O₃ remained stable, and SO₂ significantly declined due to environmental policies.
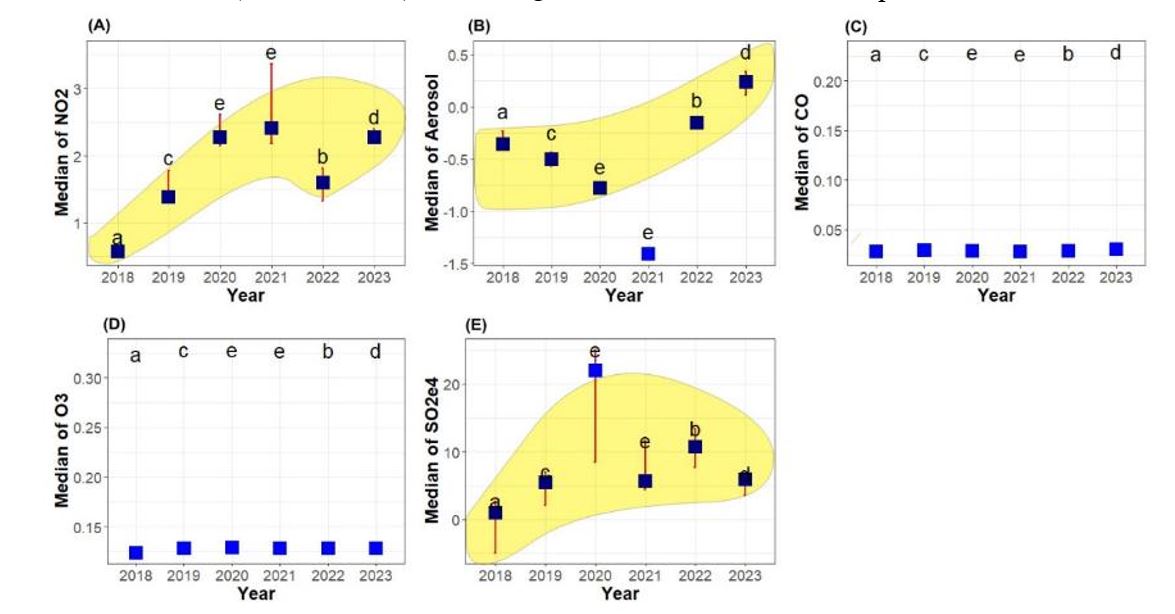
Figure 11: One-Way ANOVA results for air pollutant medians (A to E: 2018-2023)
One-Way ANOVA Analysis of Environmental Variables (2018–2023)
One-way ANOVA results revealed significant temporal variations in environmental factors from 2018 to 2023 (Figure 12).
NDVI (A): Increased in 2019 and 2021, indicating improved vegetation health, but declined in 2022 and 2023, suggesting reduced vegetation cover.
Soil Temperature (B): Remained stable from 2018 to 2022, with a notable rise in 2023, possibly due to climatic or land-use changes.
Surface Runoff (C): Peaked in 2021 and 2022, likely due to increased precipitation, and then declined in 2023, reflecting shifts in rainfall or land management.
Temperature at 2 Meters (D): Showed a consistent trend with spikes in 2021 and 2023, potentially affected by climatic fluctuations.
Wind Speed (E): Increased gradually from 2018 to 2023, indicating evolving meteorological conditions.
Total Precipitation (F) peaked in 2020 and 2021, followed by a decline in 2023, suggesting drier conditions or shifting weather patterns.
These results underscore significant interannual variability, highlighting the need for continuous monitoring and adaptive environmental management.
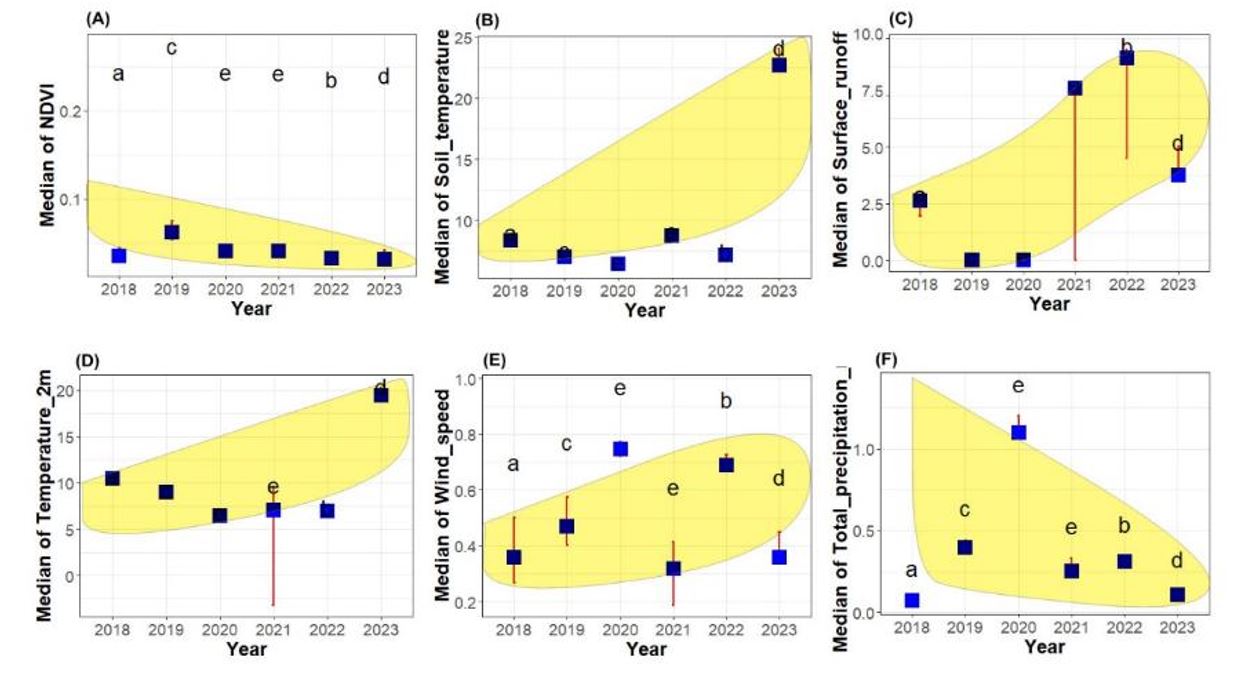
Figure 12: One-Way ANOVA results for environmental variables medians (A to F: 2018-2023)
Temporal Trends in Pollution Variables (2019–2023)
The graphs illustrate pollutant concentration trends from March 2019 to November 2023 (Figure 13).
NO₂ (A): Showed a significant increase, with peaks in summer (June and September), likely due to higher vehicular and industrial emissions.
UV Aerosol Index (B): Minor fluctuations were observed with a slight upward trend, indicating stable aerosol pollution with seasonal variations.
CO (C): Remained relatively stable with occasional peaks, suggesting steady pollution levels with periodic fluctuations;
O₃ (D): Exhibited a cyclical pattern, with higher concentrations in summer, driven by photochemical reactions and higher temperatures.
SO₂ (E): Mostly stable with annual peaks, possibly linked to seasonal industrial activities or heating.
Key Insights
Seasonal variations significantly affect pollutants such as NO₂, O₃, and SO₂, with peaks in the warmer months. While CO and the UV Aerosol Index remained stable, NO₂ and O₃ showed pronounced seasonal cycles.
Implications
Understanding these trends can help in designing seasonal pollution control measures and targeted policies to effectively improve air quality.
Figure 13: Trends in pollutant concentrations (NO2, Aerosol, CO, O3, and SO2) from March 2019 to November 2023
Discussion
Environmental and Pollution Trends in Yazd-Ardakan Plain (2019–2023)
Environmental monitoring and pollution management have become critical due to rapid industrialization and urbanization, especially in arid and semi-arid regions such as the Yazd-Ardakan plain in Iran 24. This study aimed to explore the relationships between environmental and climatic factors using remote sensing data and GEE and R software. Parameters such as temperature, pollution levels (NO ₂, CO, O ₃, SO ₂, and aerosols), precipitation, and NDVI were analyzed to understand their implications for regional climate stability and sustainable development.
Temperature and Pollution Trends
Landsat-8 data revealed a consistent upward trend in temperature across the Yazd-Ardakan plain, consistent with global research on rising temperatures in arid regions due to both natural climate variability and anthropogenic influences 25. The temperature increase is linked to rising pollution levels. Sentinel-5P data showed elevated concentrations of pollutants (NO₂, CO, O₃, SO₂, and aerosols), which correlated positively with higher temperatures, reinforcing the findings on the urban heat island effect, where pollution exacerbates temperature increases 25.
Precipitation and Vegetation Trends
Precipitation and vegetation cover showed a significant decline over the study period, aligning with the findings of Liu et al. (2018), who reported decreasing vegetation in arid regions due to reduced rainfall 26. The decrease in NDVI values in the study suggests that declining vegetation cover, which contributes to heat regulation through evapotranspiration, exacerbates the rise in temperature 27. This reduction in vegetation is consistent with the findings of Taghizadeh-Mehrjardi et al. (2020), who highlighted the negative impact of water scarcity on vegetation in Iran 28.
Regional Variations and Industrial Impact
The study found regional variations within the Yazd-Ardakan plain. Ardakan and Hasan Abad exhibited higher NDVI values, indicating healthier vegetation, likely due to better land management practices than in the industrial zones. These findings align with those of studies showing that effective vegetation management can mitigate the impacts of environmental stress 29. The highest soil temperatures were observed in the control region, likely due to industrial activities. Surface runoff was significantly higher in the Steel region, consistent with the presence of impermeable surfaces and poor water management, which is typical of industrial areas 30.
Pollution Distribution and its Effect on Climate
The spatial distribution of pollutants across regions, such as Steel, Yazd, and control, showed significant variability. The highest concentrations of NO₂ and aerosols were found in industrial zones, which aligns with the findings of Zohdirad et al. (2019) 31, who identified industrial emissions as major pollution sources. Similarly, CO and SO₂ concentrations followed a similar pattern, with elevated levels in industrial areas, highlighting the impact of local emissions on the air quality.
Climate Stability and Policy Implications
Increasing temperatures, coupled with declining precipitation and vegetation, contribute to regional climate instability, as observed by Dai and Bloecker (2019) 32. This instability has implications for water resource and land management 33. These findings emphasize the need for adaptive strategies to address environmental changes. Xiong et al. (2023) noted that declining vegetation and water availability will continue to affect climate stability unless properly managed 34.
This study confirmed both hypotheses, demonstrating that trends in temperature, air pollution, precipitation, and vegetation cover significantly affect the environmental quality and regional climate stability. By utilizing remote sensing data, GEE, and R software, the present study developed a comprehensive monitoring framework that can guide air quality management and inform policymakers for sustainable development 35.
Conclusion
This study analyzed climatic and environmental changes in the Yazd-Ardakan plain from 2018 to 2023, revealing significant trends in temperature, pollution, precipitation, and vegetation cover. The results indicated an increase in temperatures and pollutant concentrations, including NO₂, CO, O₃, SO₂, and aerosols, which were strongly correlated with decreasing precipitation and vegetation cover. These environmental shifts have worsened air quality, exacerbating the urban heat island effect and contributing to public health risks such as increased heat stress and respiratory diseases. Furthermore, the reduction in precipitation and vegetation significantly affects water resource management, compounding the challenges faced by the region’s semi-arid climate.
This study emphasizes the need for targeted pollution control and strategic environmental interventions to address these issues. Future research should build on these findings by integrating global studies and incident assessments to refine management strategies and guide policymakers. Using advanced monitoring technologies, such as remote sensing and geographic information systems, this study provides valuable insights into mitigating environmental degradation and promoting sustainable development in the Yazd-Ardakan plain and similar regions globally. The implementation of these strategies is critical for enhancing environmental resilience and ensuring long-term ecological stability.
Acknowledgements
We would like to thank all individuals and institutions that contributed to thisresearch. Their support was invaluable for conducting this study. The present study is the result of a Ph.D thesis supported by Ya.C., Islamic Azad University.
Funding Information
Private funding by authors
Conflict of Interest /Competing interests
None
Availability of Data and Material
Data are available when requested
Consent to Publish
Authors consent to publishing
Authors Contributions
All authors contributed to the manuscript. All authors read and approved the final manuscript. MAG, AAJ and SMM contributed to the Project administration, Conceptualization, Formal analysis and Writing–original draft. SAM, and MDZ contributed to the Methodology and Writing – review and editing.
Code availability
Not applicable, or for e.g. GEE code, ….
This is an Open-Access article distributed in accordance with the terms of the Creative Commons Attribution (CC BY 4.0) license, which permits others to distribute, remix, adapt, and build upon this work for commercial use.
References
1. Yang L, Luo X, Ding Z, et al. Restructuring for growth in development zones, China: a systematic literature and policy review (1984–2022). Land. 2022;11(7):972.
2. Syrek-Gerstenkorn Z, Syrek-Gerstenkorn B, Paul S. A comparative study of SOx, NOx, PM2. 5 and PM10 in the UK and Poland from 1970 to 2020. Applied Sciences. 2024;14(8):3292.
3. Manisalidis I, Stavropoulou E, Stavropoulos A, et al. Environmental and health impacts of air pollution: a review. Front Public Health. 2020;8:14.
4. Ghorani-Azam A, Riahi-Zanjani B, Balali-Mood M. Effects of air pollution on human health and practical measures for prevention in Iran. Journal of Research in Medical Sciences. 2016;21(1):65.
5. Rocha J, Oliveira S, Viana CM, et al. Climate change and its impacts on health, environment and economy. InOne Health. Academic Press: Elsevier; 2022.p. 253-79.
6. Shivanna KR. Climate change and its impact on biodiversity and human welfare. Proceedings of the Indian National Science Academy. 2022;88(2):160-71.
7. Orru H, Ebi K, Forsberg B. The interplay of climate change and air pollution on health. Curr Environ Health Rep. 2017;4:504-13.
8. Wang J, Han J, Li T, et al. Impact analysis of meteorological variables on PM2. 5 pollution in the most polluted cities in China. Heliyon. 2023;9(7).
9. Yang L, Driscol J, Sarigai S, et al. Google Earth Engine and artificial intelligence (AI): a comprehensive review. Remote Sens (Basel). 2022;14(14):3253.
10. Andreatta D, Gianelle D, Scotton M, et al. Estimating grassland vegetation cover with remote sensing: a comparison between Landsat-8, Sentinel-2 and PlanetScope imagery. Ecol Indic. 2022;141:109102.
11. Tamiminia H, Salehi B, Mahdianpari M, et al. Google Earth Engine for geo-big data applications: a meta-analysis and systematic review. ISPRS J Photogramm Remote Sens. 2020;164:152-70.
12. Moradi H, Talaiekhozani A, Kamyab H, et al. Development of equations to predict the concentration of air pollutants indicators in Yazd City, Iran. J Inorg Organomet Polym Mater. 2024;34(1):38-47.
13. Ghorbanian A, Zaghian S, Asiyabi RM, et al. Mangrove ecosystem mapping using Sentinel-1 and Sentinel-2 satellite images and random forest algorithm in Google Earth Engine. Remote Sens (Basel). 2021;13(13):2565.
14. Karam A, Rayati Shavvazi M, Ghafarian Malamiri HR, et al. Analyzing the spatial-temporal changes of landforms and land-use in the desertification process of Yazd-Ardakan plain using maximum likelihood algorithm. Journal of Geography and Environmental Hazards. 2018;7(1):17-36.
15. Liu Z, Chen Y, Chen C. Analysis of the spatiotemporal characteristics and influencing factors of the NDVI based on the GEE cloud platform and Landsat images. Remote Sens (Basel). 2023;15(20):4980.
16. Tabunschik V, Gorbunov R, Gorbunova T. Unveiling air pollution in crimean mountain rivers: analysis of sentinel-5 satellite images using Google Earth Engine (GEE). Remote Sens (Basel). 2023;15(13):3364.
17. Xing H, Zhu L, Chen B, et al. Spatial and temporal changes analysis of air quality before and after the COVID-19 in Shandong Province, China. Earth Sci Inform. 2022;15(2):863-76.
18. Lewińska KE, Ernst S, Frantz D, et al. Global overview of usable Landsat and Sentinel-2 data for 1982-2023. Data Brief. 2024:111054.
19. Wang Q, Li J, Jin T, et al. Comparative analysis of Landsat-8, Sentinel-2, and GF-1 data for retrieving soil moisture over wheat farmlands. Remote Sens (Basel). 2020;12(17): 2708.
20. Bonansea M, Ledesma M, Bazán R, et al. Evaluating the feasibility of using Sentinel-2 imagery for water clarity assessment in a reservoir. J South Am Earth Sci. 2019;95: 102265.
21. Munyati C, Balzter H, Economon E. Correlating Sentinel-2 MSI-derived vegetation indices with in-situ reflectance and tissue macronutrients in savannah grass. Int J Remote Sens. 2020;41(10):3820-44.
22. Voors R, de Vries J, Bhatti IS, et al., editors. TROPOMI, The Sentinel 5 Precursor Instrument for Air Quality and Climate Observations: Status of the Current Design. InInternational Conference on Space Optics—ICSO 2012: SPIE; 2017.p. 442-6.
23. ED Chaves M, CA Picoli M, D. Sanches I. Recent applications of Landsat 8/OLI and Sentinel-2/MSI for land use and land cover mapping: a systematic review. Remote Sens (Basel). 2020;12(18):3062.
24. Sun L, Yu Y, Gao Y, et al. Remote sensing monitoring and evaluation of the temporal and spatial changes in the eco-environment of a typical arid land of the Tarim Basin in Western China. Land. 2021;10(8):868.
25. Almalki R, Khaki M, Saco PM, et al. Monitoring and mapping vegetation cover changes in arid and semi-arid areas using remote sensing technology: a review. Remote Sens (Basel). 2022;14(20):5143.
26. Liu H, Zheng L, Yin S. Multi-perspective analysis of vegetation cover changes and driving factors of long time series based on climate and terrain data in Hanjiang River Basin, China. Arabian Journal of Geosciences. 2018;11:1-16.
27. Feldman AF, Short Gianotti DJ, Dong J, et al. Tropical surface temperature response to vegetation cover changes and the role of drylands. Glob Chang Biol. 2023;29(1):110-25.
28. Taghizadeh-Mehrjardi R, Nabiollahi K, Rasoli L, et al. Land suitability assessment and agricultural production sustainability using machine learning models. Agronomy. 2020; 10(4):573.
29. Lal R. Soil management for carbon sequestration. South African Journal of Plant and Soil. 2021;38(3):231-7.
30. He J, Su D, Lv S, et al. Analysis of factors controlling soil phosphorus loss with surface runoff in Huihe National Nature Reserve by principal component and path analysis methods. Environ Sci Pollut Res Int. 2018;25:2320-30.
31. Zohdirad H, Montazeri Namin M, Ashrafi K, et al. Temporal variations, regional contribution, and cluster analyses of ozone and NO x in a middle eastern megacity during summertime over 2017–2019. Environ Sci Pollut Res Int. 2022:1-7.
32. Dai A, Bloecker CE. Impacts of internal variability on temperature and precipitation trends in large ensemble simulations by two climate models. Clim Dyn. 2019;52:289-306.
33. Kogan F. Remote sensing land surface changes: the 1981-2020 intensive global warming: Springer; 2022.
34. Xiong J, Zheng Y, Zhang J, et al. Impact of climate change on coastal water quality and its interaction with pollution prevention efforts. J Environ Manage. 2023;325:116557.
35. G. Pricope N, L. Mapes K, D. Woodward K. Remote sensing of human–environment interactions in global change research: a review of advances, challenges and future directions. Remote Sens (Basel). 2019;11(23):2783.