Investigating the Impact of Environmental Factors on Electricity Consumption Using Spatial Data Mining and Artificial Neural Network:
A Case Study in Yazd City
Alireza Sarsangi 1, Ara Toomanian 1*, Najmeh Neysani Samany 1, Majid Kiavarz 1, Mohammad Hossein Saraei 2
1 Department of Remote Sensing and GIS, Faculty of Geography, University of Tehran, Tehran, Iran.
2 Department of Geography, Yazd University, Yazd, Iran.
A R T I C L E I N F O |
|
ABSTRACT |
ORIGINAL ARTICLE |
|
Introduction: Modeling energy demand in different energy consuming sectors is a crucial measure for effective management of the energy sector and appropriate policies to increase productivity. The rising importance of energy resources in economic development is evident. Sustainable energy use is crucial for environmental protection and social progress. Understanding the factors affecting energy consumption is essential for effective energy management. Therefore, the purpose of the current study is to investigate the impact of environmental factors on household electricity consumption in Yazd city.
Materials and Methods: In the present research, various environmental factors affecting electricity consumption, including air pollution, air temperature in homes, ground surface temperature, and green space were investigated. The effects of these factors on electricity consumption of subscribers were investigated with ANN and apriori methods.
Results: Among the environmental factors, the distance to the regional park, the area of the park, and the amount of vegetation at a distance of 300m have the greatest impact, respectively, and the average summer air temperature, the amount of vegetation at a radius of 500 m, the distance from the local park, and the average summer NDVI have had the smallest effect. Unlike neural network methods, apriori presents relationships between parameters affecting electricity consumption transparently in the form of rules.
Conclusion: It's used to identify the most frequently occurring elements and meaningful associations in a dataset. Greenspace can be a mitigation strateegy for reduction of energy consumption. |
Article History:
Received: 25 May 2024
Accepted: 10 July 2024
|
|
*Corresponding Author:
Ara Toomanian
Email:
a.toomanian@ut.ac.ir
Tel:
+98 912 2782641 |
|
Keywords:
Artificial neural network,
Space syntax,
Electricity consumption,
Remote sensing,
Spatial data mining,
Yazd City. |
Citation: Sarsangi A, Toomanian A, Neysani Samany N, et al. Investigating the Impact of Environmental Factors on Electricity Consumption Using Spatial Data Mining and Artificial Neural Network: A Case Study inYazd City. J Environ Health Sustain Dev. 2024; 9(3): 2354-68.
Introduction
Today, electricity is considered as one of the most important energy carriers of the country, an effective factor in production and a vital commodity in consumption 1. Environmental issues caused by the use of fossil fuels, which is one of the problems facing the world today, have led to an increase in the global tendency to use less polluting and healthier fuels such as electricity 2. Modeling energy demand in different energy consuming sectors is one of the crucial measures for effective management of the energy sector and appropriate policy making in order to increase productivity in this sector. Energy efficiency has always been one of the main goals of energy policy makers 3. In Iran, most of the studies conducted in the field of electricity consumption have focused on electricity consumption forecasting 4. Omidi et al. studied modeling and forecasting of electricity production and consumption in Iran between 1967-2013 and evaluated the artificial neural network method as the most accurate method for forecasting electricity consumption 5. Fatahi et al. evaluated the impact of the structure of the high electricity consumption population (a case study comparing the electricity consumption of western and eastern provinces of the country) 6. Their results showed the positive effect of urbanization in eastern provinces due to the high income of the easterners and the presence of urban facilities, as well as the residents’ use of low-consumption electrical appliances; as a result, electricity consumption has been much lower.
Undoubtedly, climate changes, especially temperature, are effective in increasing or decreasing the use of heating and cooling devices 7. Numerous studies have been conducted on the influence of climatic factors on electricity consumption 8. Salmani and Mojarad investigated the relationship between weather variables and electricity consumption and electricity demand forecasting using circulation models in west of Iran 9, 10. The relationship between climatic variables and electricity consumption in thirteen stations of the region in a 28-year period was modeled using multiple regression. The results showed that the temperature of cold and hot days and relative humidity have the most significant effect on increasing electricity consumption. The results of a similar study by Wu et al. in Australia showed that the temperature of hot days, humidity, evaporation, and wind speed had the greatest impact on electricity consumption in Australia 11. The results by Basak and Foucault‘s study showed that there was a non-linear relationship between electricity consumption and temperature, especially in countries with hot climates 12. Vine also considered climate change as an important and effective challenge on household electricity demand in the state of California 13. Zheng et al. examined spatial granularity in electricity consumption forecasting and found the use of LSTM recurrent neural network to be effective 14. Through hourly electricity consumption information, Ramos et al. clustered various consumption patterns 15. Brunen et al. also investigated the effect of family behavior, awareness, and literacy on energy consumption with regard to their expenses 16.
In human societies, development becomes possible by using more energy, and in this way, in order to achieve development, human beings change the physical, chemical, biological, social and traditional characteristics of their environment. The production, transmission, and consumption of energy has important environmental effects on the earth's ecosystem. Energy production and use policies play a central role in local and regional environmental issues. Therefore, the need to determine the complex relationship between environmental issues and energy has become more tangible. The increasing importance of energy resources in formation and growth of economic processes as well as the necessity of exploiting these resources based on environmental considerations and sustainable economic and social development highlights the issue of identifying and examining the factors affecting energy consumption. It is clearly defined that this energy provides the possibility of economic development and progress. In the meantime, the abnormal cycle developed in the form of economic growth, energy use, and environmental problems should be eliminated. The most necessary action in the initial stages is to examine the factors affecting energy consumption, mostly consumed in urban areas, in this caseelectricity. One of the most important factors is the environmental factors investigated in this research. Therefore, the aim of the current study is to investigate the impact of environmental factors on household electricity consumption in Yazd city.
Material and Method
Introducing the study area
Yazd city, the center of Yazd Province with an area of over 100 square kilometers, is located in the center of the province between 47° 22° 54° to 33° 54° 24° east longitude, and 39° 47° 31° to 51° 56° 31° north latitude. Its altitude is 1215 m. According to the 2015 census, it has a population of more than 535,000 and is one of the first 15 cities in the country in terms of population (Figure 1). In six months of the year, the temperature of this city is very hot, so that the temperature reaches 50 centigrade in the summer season, therefore, in this research, the focus is on the data estimated in the summer season, when the electricity consumption increases due to the use of cooling systems.
Figure 1: The location of study area; a) Iran, b) Yazd City
Data and descriptive statistics
A: Electricity consumption data
Data about electricity consumption of Yazd city subscribers was obtained from the province's electricity distribution company for the years 2016 to 2019. The information provided includes the data of more than 350,000 electricity subscribers in 2-month withdrawals. The information collected was at the zip code and parcel level.
B: Satellite images
In the present study, the images of Landsat 8 satellite were used. Landsat 8 was launched as part of the LDCM data continuity mission on February 11, 2013. This satellite carries OLI and TIRS sensors. With the help of two bands in the atmospheric windows of 10.6 to 11.2 μm for band 10 and 11.5 to 12.5 μm for band 11, the TIRS sensor is able to record thermal infrared radiations with a spatial resolution of 100 meters 17-19. The fact that Landsat 8 is equipped with two thermal bands has distinguished it from other satellites in the Landsat series.
C: Air temperature in houses
The current research was conducted using an automatic thermometer that has the ability to measure and store the temperature in the desired sequence, at 60 points with proper distribution in the entire study area. Using these thermometers, the air temperature in home was measured hourly.
D: Air pollution
To evaluate the impact of pollution, the amount of PM10 was measured in 85 points in the courtyards of the houses.
Data processing
A parcel is described as the main cell of urban design structures, which determines the shape of the surrounding road network and the structure of internal buildings. Urban parcel data is one of the cornerstones of contemporary urban planning. In the present study, first, urban parcel data related to Yazd city was obtained from the municipality, which included 229,571 parcels. Since the aim of the current study is spatial data mining of electricity consumption, it is necessary to consider data as the basis of spatial distribution and other descriptive information and quantitative parameters to be placed on the location. Therefore, urban parcel data was used as the basis of spatial data. All urban parcels are not made up of residential houses and some include unbuilt land, government centers, schools, etc. The data on electricity consumption of urban parcels was obtained from Yazd Electricity Distribution Company. This data is recorded in a period of two months from the beginning of the solar (Persian) year.
Therefore, in this research, there were limitations in preparing electricity data because there was no access to information in time series with less sequence. In studies of electricity consumption monitoring in developed countries, hourly information on peak electricity consumption has been used. The number of electricity subscribers, in addition to directly affecting electricity consumption, indicates the number of people who use the public electricity grid to meet their needs. The presence of a large number of electricity subscribers can increase the electricity load in power plant network, and this may be due to the simultaneous use of electrical equipment at a certain time.
Algorithms
A: Spatial data mining
Data mining is considered as an intelligent data processing tool in order to understand the structures, patterns, and connections between large and complex data sets and to take advantage of the knowledge in data 20. The problem of exploring alternating item sets was first presented by Agrawal in 1993 in the form of exploring association rules among item sets 21.
Association rules are one of the main techniques in the field of data mining that can be used in different applications. It is also a method of data mining and is used to extract useful patterns from huge databases ; they are descriptive and unsupervised data mining methods, which search the data set to find the relationship between features. In fact, this method studies features associated with each other, while reducing the relationship between these features 22. These rules show the interdependencies between a large set of data items 23.
In this study, the problem of association rules is expressed as follows: I = {i1, i2, ..., im} is a set of data items, and T = {i1, i2, ..., tn} is a set of transactions, each of which includes data items from the set of data items I. Therefore, each transaction ti consists of a set of data items; so that tj ≤ I . If X and Y are assumed to be data items, the semantic association rule is of the form X → Y where , X < I ، Y < I , , and X ∩ Y = ϕ 24. In association, the rule that is in the form X → Y is called "precedence", and Y is called "result". It is clear that the value of the antecedent includes the value of the result. The range of support for the rule and the level of confidence in the rule are the most important qualitative criteria for evaluating the interestingness of the rule.
B: Apriori
So far, various algorithms have been presented to explore association rules, which differ in how to discover frequent items. The most famous algorithm in exploring association rules is the apriori algorithm 25. Apriori is a data mining method used to identify and extract relationships, structures, and patterns between items that occurr simultaneously in a database but are not clear 26. In apriori algorithm, association rules are extracted based on three indicators including confidence, support, and lift. In fact, each rule is evaluated through these three indicators, and the effective rules are selected from the set of possible rules. Support is estimated as follows Equation 1:
Where, A and B are two different data types in the database, and X is the total number of items in the database. This rule extracts all the transactions in which there are two data items A and B and compares them with the minimum value of the rule's support range specified by the user, and then, only selects transactions. Make sure that the support range of their rule is greater than or equal to the minimum support range of the rule, and the rest of the transactions are non-applicable rules that are deleted. The confidence component of the rule is calculated as follows Equation 2:

First, transactions containing data type A are calculated, and transactions containing data type B are extracted from them. Then, a comparison of the output of this relationship with the minimum level of confidence in the rule will be carried out. The lift component greater than 1 indicates a positive correlation that infers the occurrence of data B in the occurrence of data A ,and is estimated as follows Equation 326:
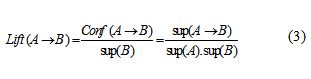
C: Artificial neural network
Artificial Neural Networks (ANNs) created by modeling the human body are composed of cells connected to each other, just like the human body. One of the most widely-used artificial neural networks in modeling and forecasting is the multilayer perceptron or MLP neural network. The MLP network consists of several layers of input, output, and hidden, where the output of the first layer is the input vector of the second layer. Similarly, the output of the second layer forms the input vector of the third layer. The outputs of the second layer show the real response of the network. In the multi-layer perceptron neural network, data processing is carried out by the activation function or the transfer function. The basic question is with what strength and quality should a neuron transmit the signal to the adjacent neuron. Adjusting neural network parameters is the main issue in model design.
Statistical analysis
In this section, the results of using multi-layered perceptron neural network for modeling and predicting electricity consumption are presented. First, the architecture of multilayer perceptron neural network is explained: this architecture includes three layers: input, hidden and output. The activation function used in the hidden layer is the sigmoid tangent function. In addition, the number of neurons in the hidden layer was 5. For network training, the Levenberg-Marquardt algorithm was chosen as the training function, which is usually the fastest and best algorithm for network training problems. For data preprocessing, two functions named "removeconstantrows" and "mapminmax" were applied to inputs and outputs. The "removeconstantrows" function was used in the data preprocessing stage. The purpose of this function is to remove columns whose value is the same in all rows and have a fixed value. These columns usually do not provide useful data for training the network, and removing them can lead to improved network performance. The "mapminmax" function is also used for input and output data and is used to normalize the data. Using this function, data values are converted to a specified range. For example, by applying this function to the data, input and output values are converted to the range between 0 and 1; this makes the influence of variables on the performance of the network more balanced and improves the performance of the trained network. The use of these two pre-processing functions is aimed at improving the quality and efficiency of the neural network. By applying these changes, data is presented to the network more readily, and the network can better learn patterns and meaningful relationships between inputs and outputs. This is more important when there are different scales and ranges for variables, inputs, and outputs, and they should be transfered to a common range so that the network is properly trained. Moreover, the number of epochs or training courses of the network was considered 200 iterations. Also, the goal of stopping the network was estimated to be 10-9