Modeling of PM10 Particulate Matter in Ahvaz City Using Remote Sensing and Meteorological Parameters
Morteza Abdullatif Khafaie 1,2, Mona Saeidi 3, Shahin Mohammadi 4, Hossein Marioryad 3, Arsalan Jamshidi 3*
1 Environmental Technologies Research Center, Medical Basic Sciences Research Institute, Ahvaz Jundishapur University of Medical Sciences, Ahvaz, Iran.
2 Department of Public Health, School of Health, Ahvaz Jundishapur University of Medical Sciences, Ahvaz, Iran.
3 Department of Environmental Health Engineering, Faculty of Health, Yasuj. University of Medical Sciences, Yasuj, Iran.
4 Department of Remote Sensing and GIS, Faculty of Earth Sciences, Shahid Chamran University of Ahvaz, Ahvaz, Iran.
A R T I C L E I N F O |
|
ABSTRACT |
ORIGINAL ARTICLE |
|
Introduction: In recent years, remote sensing (RS) products have emerged as effective tools for monitoring air pollution. This study aims to predict the concentrations of particulate matter with a diameter smaller than 10μm (PM10) using a multivariate linear regression (MLR) model, incorporating both Aerosol Optical Depth (AOD) products and meteorological parameters.
Material and Methods: In this study, data on PM10 concentrations, Aerosol Optical Depth (AOD), and meteorological parameters (wind speed, temperature, humidity, and horizontal visibility) were used. The study focused on the time 15:00 each day, as this time was identified as having significant data relevance. The methodology section also consisted of three steps: 1) pairwise correlation analysis: The relationship between meteorological parameters, AOD, and PM10 was assessed using the pairwise correlation method. 2) Model development: A MLR model was developed to predict PM10 concentrations. 3) Validation: The model was validated using a separate dataset, ensuring that 70% of the data was used for training, and 30% for testing and validation.
Results: The pairwise correlation analysis revealed a strong correlation (0.86) between AOD remote sensing index and PM10. The highest correlation (0.9) was observed during the spring season. The five developed equations to estimate the PM10 index yielded correlation coefficients ranging from 0.86 to 0.90. Notably, the highest correlation was achieved when AOD data and all the meteorological parameters were utilized simultaneously. These results highlighted the utility of remote sensing products and meteorological data in air quality monitoring and prediction.
Conclusion: This study demonstrates that a MLR model incorporating AOD and meteorological parameters can effectively predict PM10 concentrations in Ahvaz City, particularly during dust storms in hot seasons. These findings can aid policymakers and public health officials in developing strategies to mitigate the adverse effects of dust storms on air quality and public health. |
Article History:
Received: 13 June 2024
Accepted: 10 August 2024
|
|
*Corresponding Author:
Arsalan Jamshidi
Email:
Jamshidi_a@yahoo.com
Tel:
+98 9171417107 |
|
Keywords:
Air Pollution,
Remote Sensing,
Multivariable regression models,
PM10 ,
Particulate matter,
Ahvaz City. |
Citation: Saeidi M, Mohammadi Sh, Marioryad H, et al. Modeling of PM10 Particulate Matter in Ahvaz City Using Remote Sensing and Meteorological Parameters. J Environ Health Sustain Dev. 2024; 9(3): 2304-17.
Introduction
The issue of air pollution is one of the most important problems that humans have ever faced. The effects of air pollution on the environment and human health have been extensively studied in various countries, leading to increased public awareness1, 2. Emitting PM10 particles into the atmosphere, resulting from natural sources and human activities, can harm respiratory health3. The most essential human need is air, and any disruption in its quality severely impacts our ability to sustain life 1.
Ahvaz has witnessed a significant amount of dust phenomena in the past two decades, resulting in a noticeable decrease in air quality 4. Air pollutant criteria are PM2.5, CO, NOx, SO2, O3 and PM10 5. The primary health impacts of airborne particles include lung cancer, ischemic heart disease (IHD), chronic obstructive pulmonary disease (COPD), asthma, and stroke. Fine particles with a diameter of 2.5 μm or less (PM2.5) are particularly harmful, as they can penetrate deep into the lungs and reach the alveoli 6. PM10 also refers to suspended particles in the atmosphere originating from natural and human sources that considerably impact weather, the environment, and human health 7, 8. Natural sources include dust storms, jungle fires, pollen, and natural gas leakage, while human-made sources are formed as a result of outgoing gases from power plants, industries, and vehicles reacting in the air 9. Particles smaller than 10 micrometers in diameter (PM10) are introduced as the main index of suspended materials in the air as they can enter the lower respiratory system 1, 9. These particles play a major role in increasing respiratory failures, cardiovascular and pulmonary diseases, chronic bronchitis, premature mortality, and cancer. They can also reduce visibility and cause eye, ear, nose, throat, and skin irritation10. In addition to its effects on physical health, recent studies suggest that air pollution may also negatively impact brain and mental health, potentially contributing to cognitive decline, neurodegenerative diseases, and Alzheimer's disease 11, 12.
Iran is situated in a region severely affected by dust storms, owing to its location within the world's arid and semi-arid belt. This geographical positioning results in continuous exposure to both local and regional dust sources13, 14. The frequency and intensity of these storms have increased in recent years. Recent studies have shown that numerical weather prediction models are insufficient to track and diagnose dust storms automatically. Therefore, the use of remote sensing (RS) data has become necessary. RS techniques have made tremendous progress in studying and predicting trends and modeling changes in atmospheric phenomena such as dust. An effective method to investigate PM10 particles on the ground is to use statistical models and remote sensing data such as aerosol optical depth (AOD) 15, 16, which is mainly performed in urban areas with poor air quality 5, 17, 18. Given that the relationship between AOD and PM10 particles varies spatially and temporally, it is better to evaluate the relationship between these two parameters in different areas and seasons16, 19.
AOD is one of the most important parameters in the study of dust 15, 20. This parameter can be obtained from different remote sensing products such as Multi-Angle Imaging Spectroradiometer (MISR), Visible Infrared Imaging Radiometer Suite (VIIRS), and Moderate Resolution Imaging Spectroradiometer (MODIS) using an empirical model developed 21. So far, many studies have been conducted to estimate the concentration of suspended particles using satellite imagery 22. However, the use of MODIS has positive advantages due to its measurements every two days, moderate resolution, and long-term results (from 2000 for Terra and 2002 for Aqua) 23. Therefore, given the adverse effects of air pollutants, especially PM10 particles, on human health, evaluating these pollutants in polluted cities is very important 24. In this regard, various studies have been conducted regarding the comparison of PM10 particles from ground measurement stations and satellite remote sensing imagery, some of which are referred to below:
Rangzan et al. (2022) conducted a study to evaluate the spatial and temporal concentrations of PM2.5 in Khuzestan province, and investigated the factors affecting these concentrations, including wind speed, land surface temperature, and precipitation [1]. Their results showed that due to population density and the number of industries, the southern and central parts of Khuzestan have a higher potential for dust compared with the northern parts. Gharibzadeh et al. (2022) carried out a study to estimate the surface concentration of PM2.5 and PM10 using multivariate linear and nonlinear models based on remote sensing data and meteorological variables in Ahvaz 25. The results of their study showed that PM10 concentration was better predicted by multivariate nonlinear regression compared to the linear model. Solgi et al. (2022) performed a study entitled "Prediction and modeling of daily suspended PM2.5, PM10 in Hamadan’s winter using a multilayer perceptron artificial neural network (MLP)"26. The results indicated that climatic parameters such as wind and precipitation significantly reduce air pollution. Asrari et al. (2022) conducted a study to investigate and model the concentration of carbon monoxide, ozone, and PM10 in Karaj’s air using an artificial neural network 27. The results showed that the highest PM10 particulate concentration occurs in the summer and the lowest PM10 particulate concentration in the fall. Gholizadeh et al. (2022) conducted a study to evaluate the correlation between PM10 data from Sanandaj ground station and AOD data 28. The results suggested that MODIS products are suitable and practical for exploring PM10 values and their thermal patterns over extensive dust storm coverage. Damascena Aline Santos et al. (2019) studied the relationship between high-resolution AOD and particulate matter surface concentration in the São Paulo urban area 29. The results showed that populations living in urban areas are more affected by air pollution. Carmona et al. (2021) evaluated MODIS high-resolution AOD data and surface data using a group modeling approach to assess PM2.5 spatial and temporal distributions 5. The results demonstrated the best performance for seasons with calm to relatively calm winds and low to mild temperatures (winter and spring) and the worst performance in summer. Viñas Mary Joy et al. (2022) predicted the PM2.5 and PM10 air quality index using artificial neural networks 30. The results showed that AQI prediction will be suitable for predicting pollutant concentrations. Kujawska Justyna et al. (2022) applied machine learning methods to predict PM10 concentration in Lublin, Poland 31. The results showed that ANN models can estimate PM10 using data from weather stations. In another study, Plocoste Thomas et al. (2023) predicted PM10 concentration in the Caribbean region using a machine-learning model 32. The results revealed that air temperature and precipitation strongly influence African dust deposition.
The geographical location of Ahvaz, situated on the border with Iraq, and the presence of wetlands and plains in the region contribute to the existing pollution and dust storms1, 33. In addition to industrial and technological advances, the presence of power plants, a high population density, an increase in vehicles, fossil fuel consumption, oil and gas wells in the vicinity of Ahvaz, and ultimately, poor management have contributed to the transformation of this city into one of the megacities with a high air pollution coefficient14.Ahvaz is one of the most polluted cities in the country in terms of the PM10 index; thus, its air pollution has been assessed. In light of the intricate behavior of dust and the limited amount of research in Iran, it is imperative to investigate this issue. In light of the adverse effects of air pollutants, particularly PM10 particulate matter, on human health, evaluating these pollutants in polluted cities such as Ahvaz is of great importance. Therefore, the present study aims to model PM10 particulate matter using remote sensing and meteorological parameters in Ahvaz city.
Materials and Methods
Study area
Ahvaz is a historical metropolis in southwestern Iran and the capital of Khuzestan province 34. With a population of over 2.1 million, this city covers an area of 220 km2 at the geographical location of 31 degrees and 20 minutes’ north latitude and 48 degrees and 40 minutes east longitude. Figure 1 shows the location map of Ahvaz city in Khuzestan province and air pollution monitoring stations (Naderi and General Department) located in this city. One of the serious problems of Ahvaz, which is unique to this city compared to other major cities in the country, is the existence of numerous highly polluting factories within the city35.
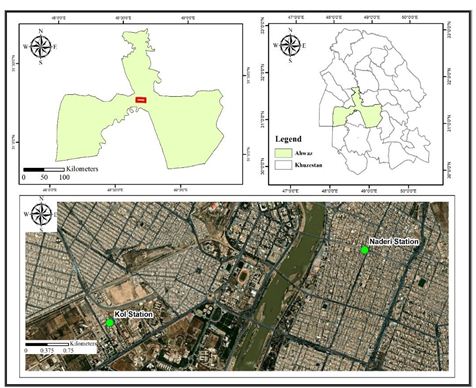
Figure 1: Location of air pollution monitoring stations in Ahvaz city.
Data used
The ground data used in this study was divided into three categories: climatic from weather stations, air pollution monitoring data, and remote sensing data, which are explained in order:
Since climatic parameters, directly and indirectly, affect PM10, climatic variables (such as temperature, wind and air humidity) can be used to investigate PM10 concentration and modeling (Asuguwa et al., 2023). So, in this study, horizontal visibility (HV), temperature (T), wind speed (WS), and air humidity (AH) for the year 2022-2023 were collected from Iran Meteorological Data Request System (www.irimo.ir) at 15:00 among other parameters.
- Ground station pollution data
In this study, the air pollution data from ground stations were obtained from the country's air pollution monitoring website (www.aqms.doe.ir). The location studied in this study belongs to an Ahvaz air quality control station (Khuzestan Environmental Protection General Department station) specified in Figure 1. The data used in this study were collected from this station in 2022-2023, totaling 219 observations. The statistical characteristics of this data for the hours and days studied are presented in Table 1.
Table 1: Statistical characteristics of PM10 (μg/m3) data for 2022- 2023 in Ahvaz city.
Station name |
Mean |
Median |
Standard deviation |
Maximum |
Minimum |
Environmental Protection
General Department |
180.16 |
115.17 |
260.72 |
2249.67 |
20 |
One of the most important factors in air pollution monitoring through RS is the use of the most suitable, easiest, and most cost-effective free satellite imagery 36. MODIS sensor can be considered an optimal choice for measuring pollution levels, especially suspended particulates 37, due to its numerous spectral bands, daily Earth imaging, and free and accessible imagery. Since the overpass time range of the MODIS sensor over Iran is between 14:00 and 15:00 and preliminary data analysis shows the highest correlation of AOD at 15:00, the focus of relating this sensor's data with ground data has been at this hour. In this study, AOD data from the MCD19A2 product was used 38, 39. The MCD19A2 dataset is a combined dataset from the Aqua and Terra satellites utilizing the Deep Blue algorithm 29, 37. This combination helps increase the spatial accuracy of the resultant product, with the extracted product's spatial resolution estimated at 1 km 29. The required data for this satellite was downloaded from NASA Earth Observation Data (www.earthdata.nasa.gov). The statistical specifications of the AOD data for different periods are presented in Table 2.
Table 2: Statistical specifications of AOD data for 2022-2023 in Ahvaz city
No. |
Period |
Mean |
Median |
Standard deviation |
Minimum |
Minimum |
1 |
Spring |
0.42 |
0.28 |
0.59 |
3.34 |
0.06 |
2 |
Summer |
0.45 |
0.36 |
0.36 |
2.25 |
0.082 |
3 |
Autumn |
0.2 |
0.21 |
0.12 |
0.82 |
0.121 |
4 |
Winter |
0.21 |
0.21 |
0.09 |
0.43 |
0.05 |
5 |
Daily |
0.36 |
0.27 |
0.41 |
3.3 |
0.05 |
Modeling using Multivariate Linear Regression (MLR)
Linear modeling using AOD can be useful in air pollution science 40. A simple trend for building a linear model is to first collect AOD data from MODIS sensor, PM10 data from ground station, and meteorological parameters. Next, the data is statistically analyzed in the second step to identify patterns, relationships, and correlations between PM10, meteorological parameters, and AOD. In the third step, based on the data analysis, which parameters are included in the modeling according to their correlation level with the dependent variable PM10. Finally, in the fourth step, the model is constructed. Equation 1 presents a simple MLR model with its parameters:
𝑌 = 𝐶 + 𝛽1𝑋1 + 𝛽2𝑋2 + ⋯ + 𝛽𝑛𝑋𝑛 |
(1) |
Where Y is the independent parameter equal to the PM10 index, the variables X1 to Xn are the meteorological parameters and AOD, respectively, and β1 to βn are the coefficients of the parameters. C is also the constant value of the model. Finally, after modeling, it is necessary to evaluate the accuracy of different models based on error indices. The Pearson correlation coefficient was used in this study, which is fully explained below.
Accuracy assessment
In this study, the accuracy of different models will be evaluated based on t Pearson regression error index (R). For this purpose, the regression coefficient between the real PM10 values from the Department of Environment station and the estimated PM10 values from meteorological data and AOD data using the MLR model was extracted using Equation 2 41.
(2) |
R=1-Σⅈ = 1Nyi-yii = 1Nyi-yi |
In this equation, R is the correlation coefficient between the two parameters, yi is the real PM10 value, ŷi indicates the estimated value using MLR models, and 𝑦̅𝑖 is the mean of the real PM10 values.
Results
This section investigates remote sensing AOD data with PM10 across different seasons. Subsequently, the results of the correlation between the parameters employed in this study will be discussed. Subsequently, several multiple linear regression (MLR) models developed using the investigated parameters will be presented. Finally, the results of the comparison between the simulated values and the actual PM10 index values will be presented.
Investigating the relationship between AOD data and PM10
The correlation results related to the PM10 index with remote sensing AOD data at 3 pm are shown in Figure 2. According to this study, AOD values and PM10 index at 3 pm indicated an annual correlation of 0.86. Moreover, the highest correlation between the different seasons was between spring and summer, with values of 0.9 and 0.86, respectively. On the other hand, the lowest correlation was related to the autumn and winter, with values of 0.13 and 0.079, respectively.
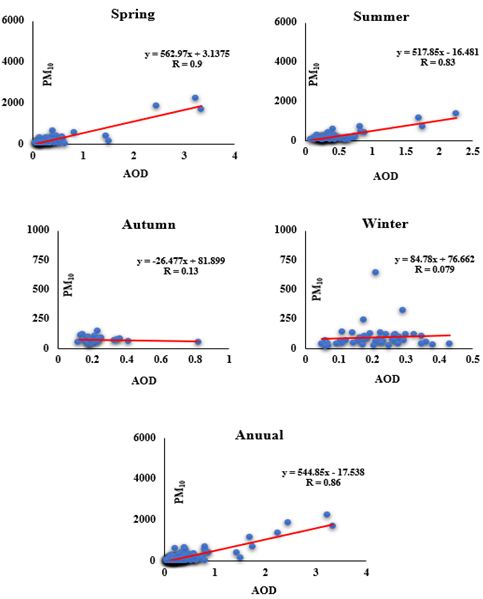
Figure 2: Regression relationship at 15:00 between MODIS sensor AOD and ground data in 2022-2023
The frequency of dusty days varies across regions, largely influenced by local climatic conditions. This phenomenon is particularly pronounced in arid and desert areas42. Several factors contribute to the occurrence of dusty days, including strong winds, decreased moisture, changes in topography, and natural processes35. Figure 3 shows the number of dusty days in Ahvaz city for the year 2022 across seasons.
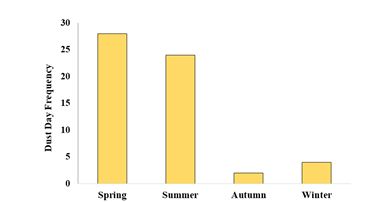
Figure 3: Dusty days in Ahvaz city during different seasons of the year 2022-2023
Evaluation of pairwise correlation of parameters
Table 3 displays the pairwise correlation of parameters in this study. In Table 3, green indicates a positive correlation between the variables, while red signifies a negative and significant correlation between the variables under study. Moreover, the color yellow was used to represent a weak relationship between the variables. The results showed that among the used parameters, the PM10 index had the highest correlation with AOD and HV, with values of 0.86 and 0.52, respectively, representing a direct relationship with AOD and an inverse relationship with HV parameter.
Table 3: Pairwise correlation relationship of parameters used in this study between pollutant PM10 and AOD and also meteorological parameters HV, WS, T, AH in Ahvaz city
Parameters |
PM10 |
HV |
WS |
T |
AH |
AOD |
PM10 |
1.00 |
- 0.52* |
0.10 |
0.17* |
- 0.20* |
0.86* |
HV |
- 0.52* |
1.00 |
- 0.03 |
0.11 |
- 0.26* |
- 0.50* |
WS |
0.10 |
- 0.03 |
1.00 |
- 0.13* |
0.01 |
- 0.01 |
T |
0.17* |
0.11 |
- 0.13* |
1.00 |
- 0.83* |
0.18* |
AH |
- 0.20* |
- 0.26* |
0.02 |
- 0.83* |
1.00 |
- 0.12* |
AOD |
0.86* |
- 0.50* |
- 0.01 |
0.18* |
- 0.12* |
1.00 |
* Correlation was significant at 95% level
Regression models for estimating PM10
After examining the pairwise correlation of the variables used, models could be presented to estimate the PM10 index using multivariate regression methods. In Table 4, 5 equations are presented in order to model the estimation of PM10 concentration using meteorological data and AOD. Their correlation values were also mentioned, ranging between 0.86 and 0.90. In other words, these equations could estimate PM10 index with this accuracy using the available data. The first model presented in this table, using only AOD with an accuracy of 0.86, could estimate the PM10 index, while by employing wind speed along with the AOD remote sensing index, model 2 was presented, and the correlation value reached 0.87. Subsequently, model 3 included a combination of two meteorological parameters, wind speed, horizontal visibility, and AOD, which achieved a correlation coefficient of 0.88. Model 4, comprising AOD and meteorological parameters such as wind speed, horizontal visibility, and humidity, showed a correlation coefficient of 0.89.
Table 4: PM10 prediction using meteorological parameters and AOD in Ahvaz city.
No |
MLR Models |
R (Pearson) |
1 |
PM10-Model 1 = - 17.206 + 544.648 × AOD |
0.86 |
2 |
PM10-Model 2 = - 92.686 + 545.856 × AOD + 0.326 × WS |
0.87 |
3 |
PM10-Model 3 = 26.923 + 509.508 × AOD + 0.314 × WS – 0.012 × HV |
0.88 |
4 |
PM10-Model 4 = 156.130 + 475.599 × AOD + 0.314 × WS – 0.020 × HV – 2.047 × AH |
0.89 |
5 |
PM10-Model 5 = 425.243 + 477.864 × AOD* + 0.241 × WS* – 0.022 × HV* – 4.428 × AH* – 5.056 × T |
0.90 |
*Aerosol Optical Depth, * Wind Speed, *Horizontal Visibility, * Air Humidity
Figure 4 shows correlation plots between the estimated value (using 5 MLR models) and the actual PM10 index data. The results of this
study indicated that model 5 could be obtained by utilizing MODIS sensor AOD and meteorological data of wind speed, air
humidity, horizontal visibility, and surface temperature among the mentioned models,
which could estimate PM10 with the highest accuracy (0.90).
.JPG)
Figure 4: Evaluation of the MLR model for estimating PM10 (μg/m3) and meteorological parameters and AOD in 2022-2023
Comparison of actual and modeled PM10 index
This modeling method uses multivariate linear regression to examine the relationship between meteorological parameter values (HV, WS, AH and T) and PM10, as seen in the regression model findings of Table 4 and Figure 5. As a result, PM10 values were estimated using the meteorological parameters and AOD, allowing comparison between the actual PM10 value and the estimated value.
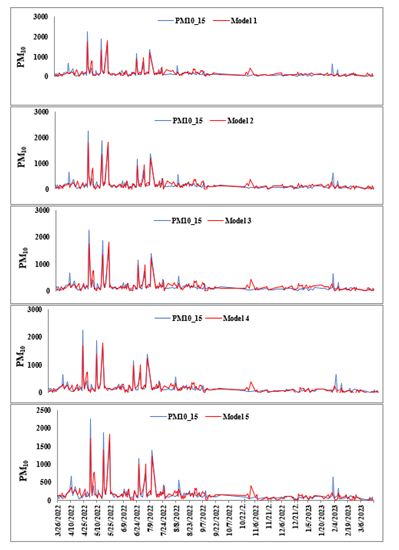
Figure 5: Comparison of actual PM10 levels with estimated values using meteorological parameters and AOD with the MLR method in 2022-2023
Discussion
The correlation at different hours with PM10 ground data may vary, but the closer it is to the ground data collection time, the stronger and better this relationship will be. Various researchers have addressed this issue, and in confirming this, one can refer to the study of Rabiei Dastjerdi et al. (2022), who used remote sensing data to estimate NO2 43; they stated that remote sensing data, if the data collection time is the same or close together, can estimate ground data with a correlation greater than 0.7. Moreover, Rangzan et al. (2023) investigated PM2.5 using remote sensing for Khuzestan province in their study and emphasized the high capability of remote sensing products in estimating air pollution indices 1.
The results of this study showed that AOD and PM10 measured on the ground at 15:00 and in different seasons exhibited differences in correlation magnitude. Given that aerosols increase as a result of higher temperature and solar radiation, and on the other hand, the intensity of surface winds increases in warm seasons, the potential for transport of larger particles are intensified. In Ahvaz, the dry periods during spring and summer contribute to the suspension of dust particles in the air. Therefore, the production and transport of aerosols in the entire atmospheric column increases, which can explain the observed AOD peak in spring.
The PM10 index in Ahvaz exhibits seasonal variation, reaching its lowest levels in winter and peaking in spring. This pattern coincides with the city's climate, where temperatures begin to rise in spring. On the other hand, autumn and winter are known as rainy seasons; thus, their AOD level is lower than in spring and summer. Additionally, cloud cover can also affect PM10 assessments, as satellite retrieval methods rely on solar reflectance, unlike ground monitoring, which measures PM10 concentration regardless of cloud conditions, requiring a clear sky. The Shin et al.’s (2019) study demonstrated that the correlation between AOD and ground-based PM10 varied with different temporal averaging and geographic locations 44. In Guo et al.’s (2017) study, similar to the present study, the best correlation was obtained with hourly remote sensing data 45. Although the highest correlation was observed with 15:00 data in this study, this may not hold for all the regions, given the location and climate. A study by Mancilla et al. (2019) stated that in summer, geological materials make up 45% of the chemical composition of coarse particles, indicating the presence of dust in the atmosphere 46. On the other hand, Damascena et al. (2021) concluded that AOD data from MAIAC provided acceptable results for predicting PM concentration using the Dark Target (DT) algorithm 29.
Additionally, the findings of this study implied that the number of days with dust particles in spring and summer was markedly higher than in winter and autumn. Hence, the main reason for the poor correlation of satellite data with ground data in winter and autumn could be the absence or low levels of dust plumes in this season. Additionally, studies by Gupta (2009) and Benas (2013) showed that with increasing temperature, especially in summer, there was a significant increase in PM10 concentration, consistent with the results of this study47, 48.
Therefore, the lowest correlation with PM10 index was observed in WS. In other words, the higher the horizontal visibility, the lower the amount of dust present in the atmosphere and the cleaner the air. On the other hand, the relationship between air humidity and PM10 index was inversely significant, indicating that increasing air humidity markedly reduced pollution levels based on this index. In fact, in warm seasons, parameters like humidity and precipitation decrease due to lower moisture, leading to reductions in the mentioned parameters and horizontal visibility, while PM10 will have higher concentrations. Moreover, the evaluation of significance levels showed that all the existing parameters (WS) had a significant correlation with PM10 index at the 95% confidence level. However, this parameter also had a positive coefficient, which could be justified by the fact that wind causes the displacement of PM10 particles. In this regard, Gupta et al. (2020) conducted a study to investigate the estimation of suspended particulate matter (PM2.5, PM10) concentration and its variations in urban areas in Bangladesh, stating that there was a significant correlation between air pollution indices (PM10 and PM2.5) and meteorological parameters 49. The review of Stirnberg et al. (2018) showed that wind speed and direction are very important in particle concentration variations. These researchers stated that the PM10 relationship with wind speed was positive, consistent with the results of this study 50.
It is noticeable that the final model, which included the results of all meteorological and remote sensing parameters, significantly enhanced the correlation coefficient and estimation accuracy. Therefore, it can be said that the simultaneous use of remote sensing data and concurrent integration with meteorological parameters can improve model accuracy. In a study by Allabakash et al. (2022) examining suspended particulate matter concentration in South Korea, meteorological parameters such as relative humidity, temperature, and wind speed were considered highly important for PM10 index modeling51. Amnuaylojaroen et al. (2022) conducted a study entitled "PM2.5 prediction in a northern Thailand urban area using multivariate linear regression model", which utilized AOD data, meteorological parameters (WS, T, and AH) and air pollutants (NO2, SO2). The results confirmed that the mean PM2.5 concentrations during the dry season is markedly higher than during the wet season. Also, the coefficient of determination values for wet and dry seasons were 0.18 and 0.26, respectively 52. Additionally, Zaman Nurul Amalin et al. (2017) studied the estimation of suspended PM10 using AOD and meteorological variables in Malaysia. The results of this study indicated that the simultaneous use of humidity, land surface temperature, and AOD data is necessary for better estimation of the PM10 index. Finally, they stated that integrating remote sensing data and meteorological parameters led to increased modeling accuracy, and the accuracy of the MLR model in estimating the daily PM10 index reached 0.81 regression 53. It should be noted that the results obtained in this study demonstrated better outcomes and higher correlations compared to other similar studies. It can be stated that the results were consistent with previous studies.
Conclusion
This paper utilized remote sensing data and meteorological parameters to model air pollution based on the PM10 index in Ahvaz city. This study first examined the correlation of AOD in different seasons with the PM10 index. The results demonstrated that the highest correlation was 0.9 in the spring, while the lowest correlation exhibited a value of less than 0.1 in the autumn. On the other hand, the pairwise correlation of parameters with the PM10 index showed that parameters like AOD, wind speed, and temperature have a direct relationship with pollution levels, while horizontal visibility and relative humidity have a significant inverse relationship with PM10 values. The results also demonstrated that AOD has the highest correlation among the parameters used, with a value of 0.86. Regarding modeling the PM10 index using MLR models, the highest accuracy of 0.9 was achieved when remote sensing AOD and meteorological parameters (WS, HV, T, and AH) were used simultaneously in the model. However, it has been shown that using AOD and meteorological parameters is beneficial in model-based applications and pollution monitoring (e.g., public health assessments and air quality index), and thus, warrants further research. Despite the highlights of this study, future research should apply nonlinear regressions, neural networks, or machine learning to model this index. This study only performed PM10 index modeling, while other air pollutants like PM2.5 could also be investigated. Furthermore, this modeling was conducted using remote sensing data and meteorological stations within the geographical boundaries of Ahvaz City. It is recommended that the effectiveness of these findings be evaluated in other geographical areas, especially metropolitan areas.
Acknowledgements
The authors would like to express their sincere gratitude to the Environmental Protection Agency of Iran, Iran Meteorological Organization, and National Aeronautics and Space Administration (NASA) for providing the valuable data utilized in this study.
Conflict of Interest
The authors declared no conflict of interests.
Funding
This research received no external funding.
Ethical considerations
This study was based on the master's thesis in Environmental Health Engineering titled "Comparison of the 10-year trend of PM10 particulate matter pollution as reported by air quality measurement stations and NASA satellite images in Ahvaz city" at Yasuj University of Medical Sciences.
Code of Ethics
The research was approved by the Ethics Committee with the code IR.YUMS.REC.1402.133. and grant number 4020078.
Authors' contributions
Conceptualization was done by Shahin.Mohammady., Mona.Saeidi.; methodology was devised by Shahin.Mohammady., Morteza.Khafaie., Mona.Saeidi.; validation was carried out by Mona.Saeidi., Shahin.Mohammady.; data curation was done by Shahin.Mohammady., Mona.Saeidi., Morteza.Khafaie., Arsalan.Jamshidi.; original Draft was prepared by Mona.Saeidi., Shahin.Mohammady. ; review and editing was carried out by Mona.Saeidi., Shahin.Mohammady., Morteza.Khafaie., Arsalan.Jamshidi., and Hossein.Marioryad; visualization was conducted by Mona.Saeidi.; and supervision was done by Morteza.Khafaie . All the authors read and agreed to the published version of the manuscript.
This is an Open-Access article distributed in accordance with the terms of the Creative Commons Attribution (CC BY 4.0) license, which permits others to distribute, remix, adapt, and build upon this work for commercial use.
Reference
1. Rangzan K, Kabolizadeh M, Mohammadi S. Assessment of spatiotemporal changes of NO2 using TROPOMI sensor in Khuzestan province, Iran. Journal of Health System Research. 2021;17(2):87-96.
2. Amanollahi J, Kaboodvandpour S. Assessment of MODIS images and data validation to measure PM10 in related to ground station data: case study: Sanandaj, Iran. Geographic Space[Internet]. 2014;14(46):129-149. Available from: https:// sid.ir/paper/91397/en.
3. Rezaali M, Fouladi-Fard R. A narrative summary of air pollution awareness: the recent modeling implications. Journal of Environmental Health and Sustainable Development. 2021; 6(1): 1175-7.
4. Rangzan K, Zarasvandi A, Abdolkhani A, et al. Modeling of air pollution using modis data: Khouzestan dust storm. Advanced Applied Geology. 2014;4(4):38-45.
5. Carmona JM, Gupta P, Lozano-García DF, et al. Evaluation of modis aerosol optical depth and surface data using an ensemble modeling approach to assess PM2.5 temporal and spatial distributions. Remote Sensing. 2021;13(16): 3102.
6. Saeedi R, Khani Jazani R, Khaloo SS, et al. Risk assessment of occupational and public exposures to airborne particulate matter arising from a subway construction site in Tehran, Iran. Air Qual Atmos Health. 2021;14(6):855-62.
7. Alimahmoodi SS, Moayeri MH, Shataee JS. Air pollution (PM10) estimation using weather data (case study: Ahvaz City). Journal Of Natural Environment (Iranian Journal Of Natural Resources) [Internet]. 2018;71(3):385-397. Available from: https://sid.ir/paper/195033/en.
8. Rezaei Rahimi N, Fouladi-Fard R, Rezvani Ghalhari M, et al. The links between microclimatic and particulate matter concentration in a multi-storey car parking: a case study Iran. J Environ Health Sci Eng. 2022;20(2):775-83.
9. Goudarzi G, Geravandi S, Saeidimehr S, et al. Estimation of health effects for PM10 exposure using of Air Q model in Ahvaz City during 2009. Iranian Journal of Health and Environment. 2015;8(1):117-26.
10. Pooyanmehr M, Khalvandpoor M. Correlation between harmful health effects attributed to the pollutant index of suspended particles (PM10) in air quality affecting biological safety: a case study in Kermanshah metropolis, Iran. Human & Environment. 2022;20(4):15-23.
11. Safari Z, Fouladi-Fard R, Vahedian M, et al. Health impact assessment and evaluation of economic costs attributed to PM2. 5 air pollution using BenMAP-CE. Int J Biometeorol
. 2022;66(9):1891-902.
12. Fard RF, Torkamani FD, Mahvi AH, et al. Health effects from particulate air pollution in one of the industrial cities of Iran. Journal of Air Pollution and Health. 2021;6(2):135-48.
13. Neisi A, Goudarzi G, Ahmadi Angali K, et al. Evaluation of suspended particles concentration (PM2.5 and PM10) and its relationship with death rate due to cardiovascular and respiratory diseases 2014-2017, in Susangard City, Khuzestan Province, Iran. Journal of Environmental Health Engineering. 2022;9(2): 145-56.
14. Arami SA, Ownegh M, Mohammadian Behbahani A, et al. The analysis of dust hazard studies in southwest region of Iran in 22 years (1996-2017). Journal of Spatial Analysis Environmental Hazarts. 2018;5(1):39-66.
15. Hoff RM, Christopher SA. Remote sensing of particulate pollution from space: have we reached the promised land? J Air Waste Manage Assoc. 2009;59(6): 645-75.
16. Carmona JM, Gupta P, Lozano-García DF, et al. Spatial and temporal distribution of PM2.5 pollution over northeastern Mexico: application of MERRA-2 reanalysis datasets. Remote Sensing. 2020;12(14):2286.
17. Gupta P, Doraiswamy P, Levy R, et al. Impact of California fires on local and regional air quality: the role of a low‐cost sensor network and satellite observations. Geohealth. 2018;2(6):172-81.
18. Van Donkelaar A, Martin RV, Park RJ. Estimating ground‐level PM2.5 using aerosol optical depth determined from satellite remote sensing. Journal of Geophysical Research: Atmospheres. 2006;111(D21).
19. Aparicio G, Gerardino MP, Rangel MA. Gender gaps in birth weight across Latin America: evidence on the role of air pollution. J Econ Race Policy. 2019;2:202-24.
20. Chu Y, Liu Y, Li X, et al. A review on predicting ground PM2.5 concentration using satellite aerosol optical depth. Atmosphere. 2016;7(10):129.
21. Park Y, Kwon B, Heo J, et al. Estimating PM2.5 concentration of the conterminous United States via interpretable convolutional neural networks. Environmental Pollution. 2020;256: 113395.
22. Hu X, Waller LA, Al-Hamdan MZ, et al. Estimating ground-level PM2.5 concentrations in the southeastern US using geographically weighted regression. Environmental research. 2013;121:1-10.
23. Bouarar I, Wang X, Brasseur GP. Air Pollution in Eastern Asia: an integrated perspective. Basel. Springer; 2017.v. 16.
24. Kabolizade M, Rangzan K. Estimation total dissolved solids and turbidity concentration in Karkheh and Dez dam and Great Karun River by using Sentinel-2 satellite images. 2019.
25. Gharibzadeh M, Abadi ARS. Estimation of surface particulate matter (PM2.5 and PM10) mass concentration by multivariable linear and nonlinear models using remote sensing data and meteorological variables over Ahvaz, Iran. Atmos Environ X. 2022;14:100167.
26. Solgi E, Parsi Mehr M. Predicting and modeling of daily concentration of particulate matter (PM2.5 & PM10) in Hamadan winter with multilayer perceptron neural network. Environmental Researches. 2023;13(26):99-114.
27. Asrari E, Rock A. Modeling of carbon monoxide, ozone and PM10 airborne pollutants in the air of Karaj using artificial neural network. Journal of Environmental Science Studies. 2022;7(1):4666-77.
28. Gholizadeh MH, Amanollahi J, Rahimi F. Assessment of correlation between PM10 data measured at ground station of Sanandaj and AOD data of MODIS sensor. Iranian Journal of Remote Sensing & GIS. 2022;14(2):51-60.
29. Damascena AS, Yamasoe MA, Martins VS, et al. Exploring the relationship between high-resolution aerosol optical depth values and ground-level particulate matter concentrations in the Metropolitan Area of São Paulo. Atmospheric Environment. 2021;244:117949.
30. Viñas MJD, Gerardo BD, Medina RP. Forecasting PM2.5 and PM10 air quality index using artificial neural network. Journal of Positive School Psychology. 2022:6863–71-–71.
31. Kujawska J, Kulisz M, Oleszczuk P, et al. Machine learning methods to forecast the concentration of PM10 in Lublin, Poland. Energies. 2022;15(17):6428.
32. Plocoste T, Laventure S. Forecasting PM 10 concentrations in the Caribbean Area using machine learning models. Atmosphere. 2023; 14(1):134.
33. Baddock MC, Bullard JE, Bryant RG. Dust source identification using MODIS: a comparison of techniques applied to the Lake Eyre Basin, Australia. Remote Sensing of Environment. 2009;113(7):1511-28.
34. Broomandi P, Rashidi Y. The effect of dust storm on the microbial quality of ambient air in Ahvaz City and dust source identification using numerical modeling. Environmental Sciences. 2018;16(2):49-64.
35. Gharibi S, Shayesteh K. Application of Sentinel 5 satellite imagery in identifying air pollutants Hotspots in Iran. Journal of Spatial Analysis Environmental Hazarts. 2021;8(3):123-38.
36. Chu DA, Kaufman Y, Zibordi G, et al. Global monitoring of air pollution over land from the earth observing System‐Terra moderate resolution imaging spectroradiometer (MODIS). Journal of Geophysical Research: Atmospheres. 2003; 108(D21).
37. Che H, Yang L, Liu C, et al. Long-term validation of MODIS C6 and C6. 1 Dark Target aerosol products over China using CARSNET and AERONET. Chemosphere. 2019;236: 124268.
38. Téllez-Rojo MM, Rothenberg SJ, Texcalac-Sangrador JL, et al. Children's acute respiratory symptoms associated with PM2.5 estimates in two sequential representative surveys from the Mexico City Metropolitan Area. Environmental research. 2020;180:108868.
39. Vu BN, Sánchez O, Bi J, et al. Developing an advanced PM2.5 exposure model in Lima, Peru. Remote sensing. 2019;11(6):641.
40. Nguyen TNT, Luu VH, Pham VH, et al. Particulate matter concentration mapping from satellite imagery. TORUS 3–toward an Open Resource Using Services: Cloud Computing for Environmental Data. 2020. p. 103-30.
41. Carmona García JM. Satellite-derived data and ground-based measurements relationships for assessing local and regional distributions of PM2.5: model development and applications.
42. Escudero M, Querol X, Ávila A, et al. Origin of the exceedances of the European daily PM limit value in regional background areas of Spain. Atmospheric environment. 2007;41(4):730-44.
43. Rabiei-Dastjerdi H, Mohammadi S, Saber M, et al. Spatiotemporal analysis of NO2 production using TROPOMI time-series images and Google Earth Engine in a middle eastern country. Remote Sensing. 2022;14(7):1725.
44. Shin M, Kang Y, Park S, et al. Estimating ground-level particulate matter concentrations using satellite-based data: a review. GIsci Remote Sens. 2020;57(2):174-89.
45. Guo Y, Tang Q, Gong D-Y, et al. Estimating ground-level PM2.5 concentrations in Beijing using a satellite-based geographically and temporally weighted regression model. Remote Sensing of Environment. 2017;198:140-9.
46. Mancilla Y, Hernandez Paniagua I, Mendoza A. Spatial differences in ambient coarse and fine particles in the Monterrey metropolitan area, Mexico: implications for source contribution. J Air Waste Manage Assoc. 2019;69(5):548-64.
47. Gupta P, Christopher SA. Particulate matter air quality assessment using integrated surface, satellite, and meteorological products: multiple regression approach. Journal of Geophysical Research: Atmospheres. 2009;114(D14).
48. Benas N, Beloconi A, Chrysoulakis N. Estimation of urban PM10 concentration, based on MODIS and MERIS/AATSR synergistic observations. Atmospheric environment. 2013;79: 448-54.
49. Gupta A, Moniruzzaman M, Hande A, et al. Estimation of particulate matter (PM 2.5, PM10) concentration and its variation over urban sites in Bangladesh. SN Appl Sci. 2020;2:1-15.
50. Stirnberg R, Cermak J, Andersen H. An analysis of factors influencing the relationship between satellite-derived AOD and ground-level PM10. Remote Sensing. 2018;10(9):1353.
51. Allabakash S, Lim S, Chong K-S, et al. Particulate matter concentrations over South Korea: impact of meteorology and other pollutants. Remote Sensing. 2022;14(19):4849.
52. Amnuaylojaroen T. Prediction of PM2.5 in an urban area of northern Thailand using multivariate linear regression model. Advances in Meteorology. 2022;2022(1): 3190484.
53. Zaman NA, Kanniah KD, Kaskaoutis DG. Estimating particulate matter using satellite based aerosol optical depth and meteorological variables in Malaysia. Atmospheric Research. 2017;193: 142-62.